Embracing Imperfection: AI in Academia – Part 2
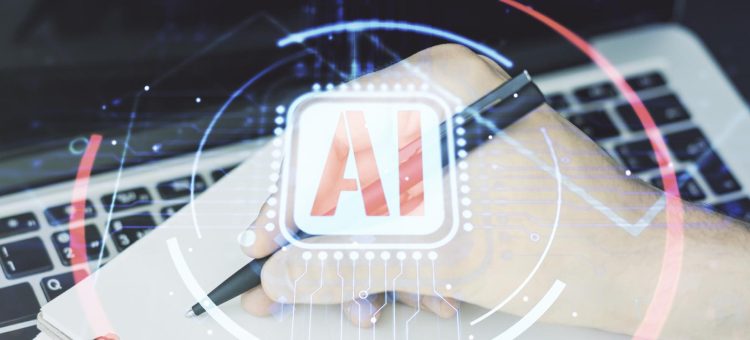
A lot has changed since we wrote our first post about academia embracing AI-assisted research and education, where we found ourselves nodding along to the thoughts of Avi Staiman and being inspired by the conversation of the leader of AI in our times. Yes, we are talking about Sam Altman, who was fired over the Thanksgiving weekend and rehired a few days after, in one of the best tech drama storylines of recent times.
That does typify the situation in AI in education and academia, where one could easily find a couple dozen stories to follow every day, and new frontiers being allegedly breached. We write this about a week after Google announced Gemini, which was hyped up to be GPT killer. We’re already getting conflicting reports on its performance, but this is a good time to evaluate why academia and education are trying hard to swim upstream in the AI progression river, without really trying to learn proper swimming technique.
Historically, we have experienced the academia being very skeptical of disruptive technologies (like the internet) before these technologies become integral. Waiting for things to become the norm might have been acceptable if the changes (read AI integration) failed to offer the type of benefit that they promise. AI integration could level things up and improve DEI, efficiency, and productivity in academia. Yet, we still seem to be hung up on hasty and arguably ill-thought-out integration.
About AI detectors
Circling back to what we started writing about in Part 1—the flawed detection of AI-generated content. As part of the roles we serve professionally, we have had the opportunity to talk to leading administrators from institutions around the world. There is a surprising variation in awareness (or lack of it) among AI-related topics among these institutions, and it is only surprising because the people who are adversely affected by the incorrect flagging are raising their voices on communities around the internet.
The main bone of contention from the individuals who are adversely affected by the false positives from AI detectors is way the decision-makers, who continue to be human, do not wish to accept the fact that no AI detection tool will be 100% accurate. At least, in the near future. Remember that the erring is the most coveted feature of the best neural network that man has ever known. Chasing perfection is futile.
Recalibrating how we use AI detectors
In our early-am crawls on Reddit, we have found an intelligent midway solution proposed consistently.
Use the existing Gen AI-based text detection tools’ results as circumstantial evidence, and let the human reviewers use all the other pieces of evidence before handing out a judgment. This sounds like the most optimal way of integrating technology that helps smoothen and enhance the human-ness that we are known for, just like AI-based spelling and grammar checks have been assisting to refine our writings for a few decades.
Then, there is the argument about whether demanding perfection from an individual student/researcher/writer (albeit with assistance from an AI tool) is necessary. This is especially relevant because the people raising their voices online about false-positive cases are generally conscientious individuals writing content by themselves but relying on AI for paraphrasing, grammar correction, and proofreading. But they have been flagged as those who cheated by the detectors.
Let us pause a moment and think about this. Does it matter that a paper is written with the help of AI but is factually robust and original in its research foundations? Second, wouldn’t it be more reasonable to take the approach that we have come to accept for plagiarism detection, where the criticality of a flag is based on the section of the manuscript where it is detected? For example, flags in the introduction sections of a manuscript are considered less critical than those in the results and discussion sections. In other words, it is the what and where that matter over the quantum of use itself.
Moving the needle forward
Further, we think that when it comes to research-advancing technology, let’s learn from product development principles. Any product or technology grows out of a fundamentally iterative process, and there will always be something to be desired. The research process is also built on iterations and evidence-based refinements. Thus, it is better to embrace emerging technology and wait for incremental improvements so that we do not miss out on the short-term benefits of early adoption while awaiting further refinements and associated long-term benefits.
Who knows, perhaps AI-generated imperfections could be utilized more creatively for providing training on critical thinking and fact-checking for humans, while human-generated imperfections can be smoothed out by the same tools. Doesn’t this sound like a more efficient way to buttress the educational and research ecosystems instead of saddling them with confusing at best, and myopic at worst, regulations?
A forward-thinking mindset, pronto
“I’ve said too much, I haven’t said enough.”
― Michael Stipe, Losing My Religion
To conclude this two-part essay, we must recognize AI’s potentially unbound potential while consciously acknowledging its imperfections, and work toward building AI-enabled/assisted research and education ecosystems. Such infrastructure should be aimed at serving the best interests of the students, educators, and the institution of science and knowledge dissemination. The integration of AI into education and research is not a question of whether it should be done but rather how and when it should be done. Let’s not wait for perfection—instead, let’s accept AI’s imperfections while using it as a valuable companion and asset.
In case you missed it, here’s part one of this essay. We are super interested in knowing what you think, so do share your opinions, and let’s see our way to an AI-enabled future.