AI’s Impact on Climate: Balancing progress and sustainability
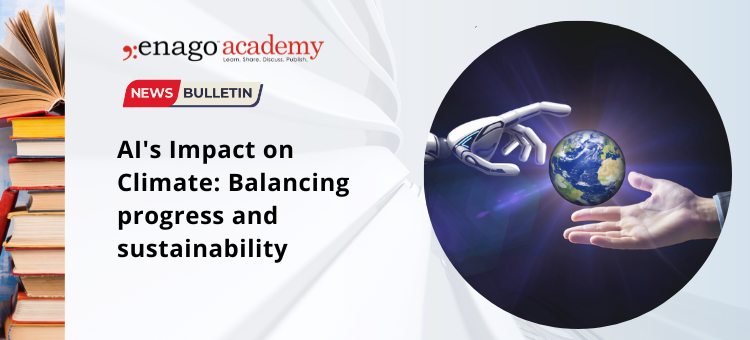
As artificial intelligence (AI) rapidly transforms various sectors of the global economy, a critical question arises: Will the widespread adoption of AI accelerate or slow down the world’s progress toward achieving net-zero emissions?
Direct and Indirect Impact of AI
Studies indicate that the current direct impacts of AI on climate are relatively small. International Energy Agency (IEA) states that AI operations for large models consume around 7-11 terawatt-hours (TWh) of electricity annually, accounting for approximately 0.01% of global greenhouse gas emissions. However, this figure is expected to grow as the demand for AI services is projected to rise by 30-40% annually over the next 5-10 years.
The indirect effects of AI on emissions are less clear but potentially more significant. Some AI applications are curated to address climate change by reducing emissions from various sectors, optimizing supply chains, and accelerating the development of new materials for renewable energy sources. However, there is also a risk of negative indirect impacts, such as cheaper fossil fuel extraction, and potential disruptions to political and economic stability, which could have adverse effects on poverty, food security, and social inequalities.
Need for AI-Driven Emissions Scenarios
Given the vast uncertainties surrounding AI’s impact, the scientific community needs to develop a set of policy-relevant scenarios to quantify the potential effects of AI expansion on emissions under various assumptions. These scenarios should range from a ‘reference’ case without widespread AI adoption to an ‘aspirational’ case where the United Nations Sustainable Development Goals are achieved.
The five essential elements for building credible AI-driven emission scenarios are:
1. Link to Existing Climate Models
AI should be integrated into the widely used Shared Socioeconomic Pathways (SSPs) and other established frameworks used by the climate community to assess future greenhouse gas emissions.
2. Develop quantitative analytical frameworks
Improved data and analytical frameworks are needed to quantify both the direct and indirect impacts of AI on emissions.
3. Open Data Sharing
Standards should be established for measuring, reporting, verifying, and disseminating AI-related data to ensure quality and accessibility. AI companies would need to share proprietary data on factors like energy use and computing demands in secure, anonymized ways to inform modelling efforts.
4. Share Frequent Updates
AI technology is advancing rapidly, necessitating annual or biannual updates to the scenarios.
5. Build Global AI-Climate Consortium
An international consortium should be established to undertake the development of AI-driven emissions scenarios, gathering specialists from various disciplines and ensuring open access to data and models.
As AI continues to enter various industries, decisions regarding its development and use must be made with sustainability in mind. By developing standard AI-driven emissions scenarios, policymakers, investors, advocates, private companies, and the scientific community will have the tools to make informed decisions regarding AI and the global race to net-zero emissions.
Financial support is essential to maintain the proposed international consortium and support the regular updating of scenarios. This could come from a combination of philanthropic, private, governmental, and intergovernmental sources. Collaborative efforts from all stakeholders will be crucial in countering the potential impacts of AI on climate change and ensuring a sustainable future.