AI-Driven Hypotheses: Real world examples exploring the potential and challenges of AI-generated hypotheses in science
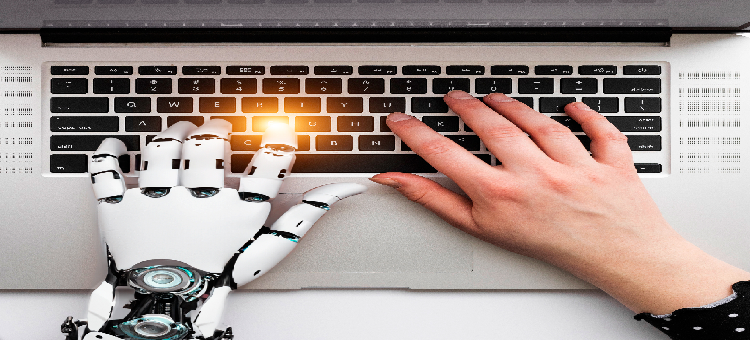
Artificial intelligence (AI) is no longer confined to mere automation; it is now an active participant in the pursuit of knowledge and understanding. However, in the realm of scientific research, the integration of AI marks a significant paradigm shift, ushering in an era where machines and human actively collaborate to formulate research hypotheses and questions. While AI systems have traditionally served as powerful tools for data analysis, their evolution now allows them to go beyond analysis and generate hypotheses, prompting researchers to explore uncharted domains of research.
Let’s delve deeper in understanding this transformative capability of AI and the challenges established in research hypothesis formation, emphasizing the crucial role of human intervention throughout the AI integration process.
Potential of AI-Generated Research Hypothesis: Is it enough?
The discerning ability of AI, particularly through machine learning algorithms, has demonstrated a unique capacity to identify patterns across vast datasets. This has given rise to AI systems not only proficient in analyzing existing data but also in formulating hypotheses based on patterns that may elude human observation alone. The synergy between machine-driven hypothesis generation and human expertise represents a promising frontier for scientific discovery, underscoring the importance of human oversight and interpretation.
The capability of AI to generate hypotheses raises thought-provoking questions about the nature of creativity in the research process. However, although AI can identify patterns within data, the question remains: can they exhibit true creativity in proposing hypotheses, or are they limited to recognizing patterns within existing data?
Furthermore, the intersection of AI and research transcends the generation of hypotheses to include the formulation of research questions. By actively engaging with data and recognizing gaps in knowledge, AI systems can propose insightful questions that guide researchers toward unexplored avenues. This collaborative approach between machines and researchers enhances the scope and depth of scientific inquiry, emphasizing the indispensable role of human insight in shaping the research agenda.
Challenges in AI-Driven Hypothesis Formation
Despite the immense potential, the integration of AI in hypothesis formation is not without its challenges. One significant concern is the “black box” nature of many advanced AI algorithms. As these systems become more complex, understanding the reasoning behind their generated hypotheses becomes increasingly challenging for human researchers. This lack of interpretability can hinder the acceptance of AI-driven hypotheses in the scientific community.
Moreover, biases inherent in the training data of AI models can influence the hypotheses generated. If not carefully addressed, this bias could lead to skewed perspectives and reinforce existing stereotypes. It is crucial to recognize that while AI can process vast amounts of information, it lacks the nuanced understanding and contextual awareness that human researchers bring to the table.
Real Concerns of the Scholarly Community Clouding the Integration of AI in Research Hypothesis Generation
Instance 1
In a paper published in JAMA Ophthalmology, researchers utilized GPT-4, the latest version of the language model powering ChatGPT, in conjunction with Advanced Data Analysis (ADA), a model incorporating Python for statistical analysis. The AI-generated data incorrectly suggested the superiority of one surgical procedure over another in treating keratoconus. The study aimed to demonstrate the ease with which AI could create seemingly authentic but fabricated datasets. Despite flaws detectable under scrutiny, the authors expressed concern over the potential misuse of AI in generating convincing yet false data, raising issues of research integrity. Experts emphasize the need for updated quality checks and automated tools to identify AI-generated synthetic data in scientific publishing.
Instance 2
In early October, a gathering of researchers, including a past Nobel laureate, convened in Stockholm to explore the evolving role of AI in scientific processes. Led by Hiroaki Kitano, a biologist and CEO of Sony AI, the workshop considered introducing awards for AIs and AI-human collaborations producing outstanding scientific contributions. The discussion revolved around the potential of AI in various scientific tasks, including hypothesis generation, a process traditionally requiring human creativity. While AI has long been involved in literature-based discovery and knowledge graph analysis, recent advancements, particularly in large language models, are enabling the generation of hypotheses and the exploration of unconventional ideas.
The potential for AI to make ‘alien’ hypotheses—those unlikely to be conceived by humans—has been demonstrated, raising questions about the interpretability and clarity of AI-generated hypotheses.
Ethical Considerations in AI-Driven Research Hypothesis
As AI takes on a more active role in hypothesis formation, ethical considerations become paramount. The responsible use of AI requires continuous vigilance to prevent unintended consequences. Researchers must be vigilant in identifying and mitigating biases in training data, ensuring that AI systems are not perpetuating or exacerbating existing inequalities.
Additionally, the ethical implications of AI-generated hypotheses, particularly in sensitive areas such as genetics or social sciences, demand careful scrutiny. Transparency in the decision-making process of AI algorithms is essential to build trust within the scientific community and society at large. Striking the right balance between innovation and ethical responsibility is a challenge that requires constant attention as the collaboration between humans and AI evolves.
The Human Touch: A crucial element in AI-driven research
Nuanced thinking, creativity, and contextual understanding that humans possess play a vital role in refining and validating the hypotheses generated by AI. Researchers must act as critical evaluators, questioning the assumptions made by AI algorithms and ensuring that the proposed hypotheses align with existing knowledge.
Furthermore, the interpretability challenge can be addressed through interdisciplinary collaboration. Scientists working closely with experts in AI ethics, philosophy, and computer science can develop frameworks to enhance the transparency of AI-generated hypotheses. This not only fosters a better understanding of the underlying processes but also ensures that the generated hypotheses align with ethical and scientific standards.
What the Future Holds
The integration of AI in hypothesis formation is an ongoing journey with vast potential. The collaborative efforts of humans and machines hold the promise of accelerating scientific discovery, unlocking new insights, and addressing complex challenges facing humanity. However, this journey requires a balanced approach, acknowledging the strengths of AI while respecting the unique capabilities and ethical considerations that humans bring to the table.
To Conclude…
The transformative capability of AI in hypothesis formation is reshaping the landscape of scientific research. But this is not possible without the collaborative partnership between humans and machines, having the potential to drive unprecedented progress. Thus, it is imperative to navigate the challenges associated with AI integration and embrace a symbiotic relationship between human intellect and AI; with which we can unlock the full potential of this dynamic collaboration and usher in a new era of scientific exploration and understanding.
Interesting article