Authenticating AI-Generated Scholarly Outputs: Practical approaches to take
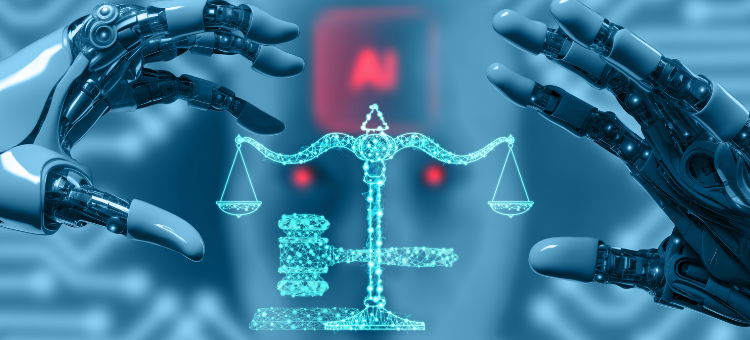
The rapid advancements in artificial intelligence (AI) have given rise to a new era of automated content generation, not sparing the scholarly publishing industry either. AI systems are now capable of generating research papers, literature reviews, data analyses, and even creative works like novels and poetry. While this technological leap offers exciting possibilities for accelerating knowledge production, it also presents a critical challenge: how can we reliably identify and authenticate outputs originating from AI systems?
As automated scholarly content generation gains traction, the need to design robust technical and policy solutions to differentiate human versus non-human contributions becomes imperative. Failing to address this issue could undermine the integrity of the academic publishing ecosystem, erode public trust in scholarly works, and raise ethical concerns about authorship and attribution.
We also took a poll to understand, which aspect of scholarly writing do researchers find AI tools to be most reliable for. Here are the results to it:
As we see researchers embracing AI tools for various scholarly writing aspects, there is a definite need to ensure that the delivered output is authentic. So here we are listing down a few practical approaches we can take to ensure the content generated is in no way disrupting academic integrity and scientific rigor.
1. Metadata Standards and Manuscript Tracking
One approach to tackling this challenge is to establish metadata standards that capture the involvement of AI systems in the content creation process. Publishers could require authors to disclose the use of AI tools during manuscript submission, and this information could be embedded within the metadata of the published work. Additionally, manuscripts could be tracked throughout the publishing pipeline, with each stage of human and AI involvement documented, providing a transparent audit trail.
2. Digital Watermarking and Fingerprinting
Another technical solution involves the use of digital watermarking and fingerprinting techniques. AI-generated content could be saturated with unique, imperceptible digital signatures or watermarks that allow for the identification of the specific AI system involved in its creation. These watermarks could be embedded within the text, images, or other components of the scholarly output, enabling verification and attribution even after publication.
3. Archivable Badges, Tags, and Micro-attributions
Building upon the concept of digital watermarking, archivable badges, tags, or micro-attributions could be assigned to AI-generated content. These visual or metadata markers would clearly indicate the involvement of an AI system in the creation process, providing transparency to readers and researchers. Such badges could be linked to the specific AI authors or models responsible for the work, allowing for proper attribution and enabling further investigation into the provenance of the content.
4. CAPTCHA for Automated Submissions
To prevent automated systems from submitting content without human oversight, publishers could implement CAPTCHA (Completely Automated Public Turing test to tell Computers and Humans Apart) or similar verification mechanisms during the submission process. These tests, designed to distinguish human users from automated bots, could serve as a checkpoint, ensuring that AI-generated content is reviewed and approved by human authors or editors before being considered for publication. However, there are likely to be some limitations—for instance, AI technologies are rapidly evolving, and digital watermarks or CAPTCHAs might become less effective over time as AI systems learn to circumvent these safeguards.
5. AI Registration Systems and Platform Agreements
Establishing AI registration systems linked to recognized institutions could provide another layer of accountability. AI systems used for scholarly content creation could be required to register with a central authority, providing details about their capabilities, training data, and institutional affiliations. This information could be used to verify the authenticity of AI-generated outputs and enable traceability.
Furthermore, platform agreements could mandate the disclosure of automation, with differentiated API keys assigned to human users and automated systems. This approach would enable publishers and content platforms to identify and manage bot versus human requests, ensuring transparency and adherence to established policies.
6. Leveraging the DOI Infrastructure
The Digital Object Identifier (DOI) system, widely used for persistent identification of digital objects, could be leveraged to capture and preserve AI provenance information. DOIs could be assigned not only to published works but also to the AI models and systems involved in their creation. This approach would enable the linking of scholarly outputs to their AI origins, facilitating attribution, version control, and the tracking of AI-generated content over time.
7. Developing Shared Community Standards and Pilots
Given the multifaceted nature of this challenge, collaborative efforts within the scholarly community are crucial. Developing shared standards, best practices, and guidelines for authenticating AI-generated content could foster consistency and interoperability across publishers, institutions, and disciplines. Pilot programs and proof-of-concept initiatives could be undertaken to test and refine proposed solutions, gathering valuable feedback from stakeholders and paving the way for broader adoption.
Other Important Gaps
Real-world case studies and examples can and are already doing a good job of illuminating the complexities of authenticating such content, offering valuable lessons and guiding the development of effective solutions. Moreover, the limitations of current technologies and the evolving capabilities of AI highlight the need for continuous innovation and vigilance in developing authentication methods. The evolution of AI ethics committees/boards seems like something we as an industry need. The traditional peer review process must also adapt to effectively scrutinize AI-generated submissions, requiring new skills and tools for reviewers. The impact of AI on scholarly communication is profound, with implications for inclusivity, diversity, and the quality of academic literature. International collaboration will also play a pivotal role in addressing the global challenges of AI in academic publishing. Feedback mechanisms for reporting and addressing suspected AI-generated content will further ensure transparency and accountability.
In conclusion, the integration of AI into scholarly publishing presents both remarkable opportunities and significant challenges. As we navigate this new frontier, the collective responsibility of authors, publishers, institutions, and the broader academic community becomes increasingly critical in maintaining the integrity and credibility of scholarly works. The ethical implications, potential biases, and intellectual property concerns associated with AI-generated content necessitate a deeper examination and thoughtful discussion. Through ongoing dialogue, experimentation, and a commitment to ethical standards, we can harness the power of AI to enhance scholarly publishing, ensuring that it continues to advance knowledge responsibly and efficiently.