Top 10 Ways to Avoid the Problem of Missing Data!
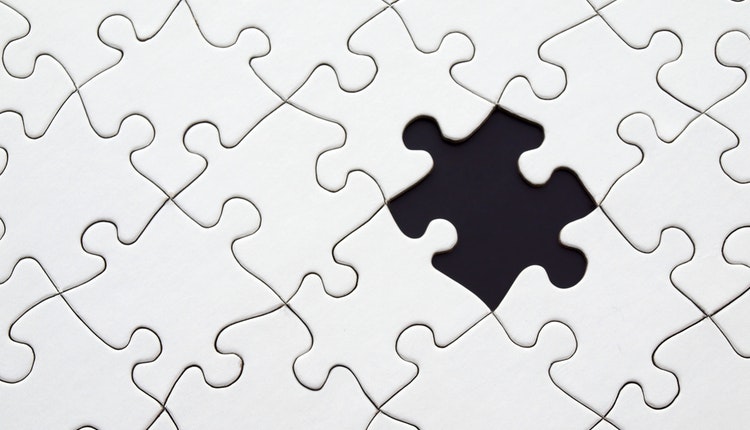
What is Missing Data?
“Missing data” is a pervasive concern amongst the entire research community! It refers to the situation where observations or information for a parameter of interest in an experimental data set is not recorded. Nearly all researchers encounter this problem at some point in their career! This can happen when participants fail to or choose not to reveal certain information during the data recording process. It may also happen if a researcher fails to design an experiment wisely and exhausts valuable but scarce experimental resources. Additionally, it might also happen when either data collection is improper, or mistakes are made during data entry.It is a real challenge when it comes to data analysis and interpretation, as there is no perfect way to deal with datasets missing crucial information or values.
The “Missing Data” Problem!
The impact of this can be serious, especially in quantitative and statistics based research as it may result in a biased estimation of crucial study parameters and poor generalizability of findings. Furthermore, overlooking missing information might lead to loss of information and in turn low statistical power due to increase in standard errors. Therefore, it is wise to identify these reasons by making plausible assumptions about the ways in which data might be missed. Researchers must create robust designs that minimize the chances of this problem.
Types of Missing Data
Types of missing data may also be classified based on the reasons or mechanisms.
Missing completely at random (MCAR)
The reasons for “missing data” are independent of the observed and missing responses, i.e. all the cases have the same probability of being missing. This is demonstrated in situation A where students were unable to take the survey due to random, unpredictable reasons.
Missing at random (MAR)
The factors that lead to missing data in this case are conditionally independent of the missing responses. For instance, missing data due to scenario B is independent of the variable of interest (i.e. whether students are facing peer pressure or not) but it might depend on other observed variable (i.e. their grades or expertise in certain extracurricular activities).
Missing not at random (MNAR)
In this case, the occurrence of incomplete data also depends on the observed data. Scenario C where students not responding to sensitive questions related to peer pressure leads to missing data problem of this category.
Example of Missing Data
Consider a situation where a researcher wants to administer a questionnaire about peer pressure in college students. On the designated date of the survey various circumstances could lead to data gone missing.
A: Some students may be absent at random due to unpredictable reasons
B: Some students may be absent as they may be representing their college in competitions or events
C: Few students may not respond accurately to sensitive questions (they might be more likely to have experienced peer pressure)
Based on this information, we might infer that data missing problem may occur at two levels – unit and/or item. A unit level missing data problem may occur if an enrolled participant fails to show up for a study or declines to take the survey. The resulting bias is known as “selective” as the responses of these participants might turn out different from those of the other participants. On the other hand, an item level missing refers to incomplete data collected from a participant enrolled in the study. For instance, the participant may miss or not answer certain questions in the survey.
How to Avoid the Missing Data Problem?
1. Design your study keeping in mind the research objectives
Ensure that you only collect data that is indispensable or absolutely essential to achieve the target objectives. This might reduce the unnecessary burden on participants and research staff of collecting non-essential information. If three assessments are sufficient to reach valid conclusions, and successfully complete the study objectives, it is not wise to conduct additional assessments. Furthermore, it could result in efficient utilization of resources (time, money, and staff) and improved quality of collected data.
2. Target an appropriate participant group
Assess the time period and inclusion/exclusion criteria before enrolling participants for your study. If your study objective is to assess the outcomes of a therapy or drug treatment for six months, it means you have to exclude participants not willing to participate for the said duration.
3. Keep your data collection protocols simple and easy to administer
Use simple words and keep your questions short and to the point. Try to be as specific as you can be. For instance, rather than asking “Do you regularly exercise?”, you may instead ask, “On an average how many days per week, do you exercise?” for obtaining objective and more precise answers.
4. Be open and flexible to different methods for data collection
Allow and make provisions for multiple methods of assessment. For instance, if the study participants are not willing to come to the survey site (clinic or research lab), allow alternative means of assessment such as self-administered questionnaires, telephonic interviews, and zoom interviews, if appropriate.
5. Documentation
Before beginning with your research, develop a detailed protocol that includes the methods for screening the participants, procedures to collect, document and record data. This will help in determining all the probable factors that could result in missing the collection of crucial information. Assess these factors thoroughly so that appropriate amendments can be subsequently made. In addition, get your study reviewed by an advisory or data monitoring committee that will scrutinize your study proposal methodically and meticulously. Their inputs will be invaluable to minimize the chances of such errors during the course of the study.
6. Communication
Conduct a training session for all participants briefing them on all aspects of the study. This might decrease the chances of participants dropping mid-way as they are now completely aware of the course of the study.
7. Trial Run
Perform a mini trial before you begin with the actual study. This might help you recognize unanticipated and unforeseen problems which are likely to occur during the course of the study. This will also help you estimate the amount of missing data you encountered in the trial run. Consequently, keeping this in view, you can perform sample size calculations. This might further reduce your chances of having an underpowered study.
8. Set prior targets
Set a limit for acceptable level of missing data. Identify the techniques that can be used to handle in case the acceptable level is breached.
9. Follow-up with participants
For clinical studies, engage and follow-up with participants to ensure they complete the entire study and provide you with all the necessary information. In case, some participants wish to withdraw from your studies, record the reasons for the same for subsequent analysis when you are interpreting the results.
10. Ensure you allocate resources that facilitate data collection effectively
Resources including travel reimbursements to participants, appropriate compensations for their participation time, salary support for the research team conducting research, funds to procure samples or reagents, etc. must be allocated and distributed wisely. A part of contingency fund must be kept reserved in case of any unforeseen events!
To sum it all, careful design considerations is one of the best ways to keep problems arising at bay! Although the problem cannot be avoided completely, the likelihood of its occurrence can be significantly minimized!
Have you faced challenges related to missing data? How did you deal with it? Let us know in the comments section below!