Can AI-Based Search Tools Replace Google Scholar?
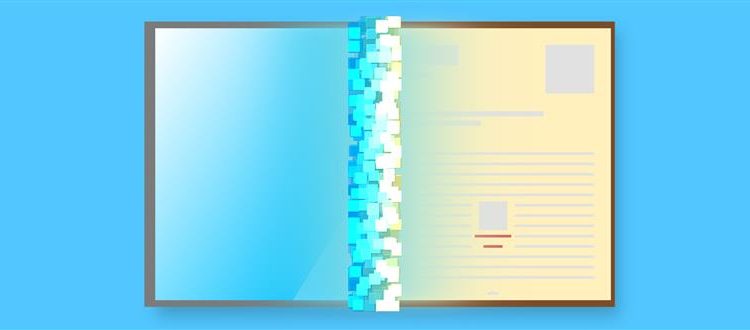
The Industrial Revolution was a time of massive innovation and machine technology. On a grand scale, machines began taking over what used to be human endeavors, bringing efficiency and mass production to the world. As with any type of innovation, there are positives and negatives. People lost their jobs to machines, which made things and distribution even easier and uniform.
AI-Based Search Platforms
We are currently witnessing a similar revolution in automation. Computers and computer-driven robots have taken over the work of many laborers. This has resulted in a loss of jobs not readily replaced by new skills and opportunities. This type of progress is, however, inevitable and has led to a more intriguing form of automation in Artificial Intelligence (AI).
AI attempts to mimic the functions of the human brain: reasoning and decision-making, interpreting sensory input, speech recognition, and more. AI has now entered the realm of academic and scientific research. AI-based search platforms attempt to follow and improve upon what human researchers do when they are searching for and trying to connect content from a variety of sources. Recently, several AI-based tools made a splash in the scientific community.
Overview of Leaders in the Field
Google Scholar is the most primitive of the latest search engines and uses keyword searches. It has access to more than 200 million articles, but cannot access metadata or citation metrics. However, scholars still find it highly useful.
Microsoft Academic improves upon Microsoft Academic Search and uses search algorithms. Its data source is accessed through an application programming interface (API) and the Open Academic Society. Powered by semantic search, it uses basic filters (author, journal, field of study), and can access 160 million publications. It is also possible to compile a leaderboard of the most cited scientists in each subdiscipline. Designers are working on a version with more personalized options.
Semantic Scholar although more advanced than Microsoft Academic in its functionality is currently restricted to the fields of computer science and neuroscience. It boasts advanced filters that can focus in on specific publications and areas of study. It can sort papers and assess citations according to relevance and importance. Paul Allen’s non-profit organization, Allen Institute for Artificial Intelligence (AI2) engineered Semantic Scholar.
Yewno distinguishes itself from other AI-based search engines by producing a graphic result from its searches. Using full-text analysis, computational semantics, neural networks, and machine-learning algorithms, Yewno makes connections among different source materials and presents them graphically using two panels: on the left is a concept map and on the right is a context bar. Located centrally on the graph, orange nodes indicate main concepts. Blue nodes indicate ideas related to the main concepts. Double-clicking on the blue nodes reveals further connections. One can also access content sources directly from these nodes. Researchers, therefore, can search according to ideas and concepts rather than keywords, making their research more specific and far-reaching.
Sparrho is another AI-based scholarly search engine that makes tremendous progress towards personalizing research. Modeled after social media platforms and news aggregation feeds, Sparrho is a science recommendation engine that combines machine and human intelligence. Its database includes journal articles, grant announcements, patents, and conference proceedings. Sparrho’s search engine uses natural language processing and can search and categorize over 45,000 journals every hour. It also tracks the interactions of its users, cataloging what they share, read, and save. Searches are highly personalized and include results from multiple disciplines, keeping researchers up-to-date in a way that is very specific to their needs.
UNSILO “provides software solutions for automated text intelligence that are used by content owners who have large collections of documents in unstructured natural language.” Since 2014, it has been Springer Nature’s choice for content enrichment. UNSILO works with partners in a variety of industries and offers content owners APIs to facilitate the extraction, connection, and distribution of content.
Competition, Progress, and Future Goals
Key players in this quest for the best content curation tools are Google, Microsoft, and AI2. Newcomers like Yewno, Sparrho, and UNSILO are proving to be innovation leaders in their ability to establish AI-based tools that appeal to researchers’ specific needs. Their goals are to satisfy a wide variety of research functions, from discovering unique connections to content management strategy.
Four key concepts for the future of AI-based search engines are personalization, specificity, efficiency, and up-to-the-minute relevancy. Researchers want to have results that are personally meaningful, specific to their area of study, efficiently delivered, and current. They also want to draw upon a wide variety of source material, ensuring that their search results are unique and have depth.
For example, Yewno for Education allows researchers to obtain multidisciplinary content in the areas of medicine, biology, physical sciences, humanities, engineering, social sciences, and they are hoping to add financial services in the near future. Owen Etzioni, chief executive at AI2, aims to have the Semantic Scholar system index all of PubMed and expand in the medical sciences.
Changes in Research Navigation
These changes in research navigation involve two seemingly contrasting concepts: globalization and personalization. Researchers have broader access to content coupled with the ability to curate that content specifically to their needs. This reflects the wider experience of the internet and social media users worldwide. Our sphere of experience has become at once global and more personal. AI-based tools of research will, no doubt, continue in the same vein.