Demystifying the Role of Confounding Variables in Research
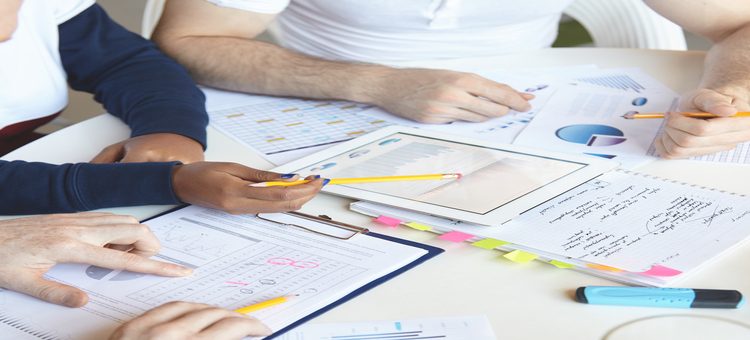
In the realm of scientific research, the pursuit of knowledge often involves complex investigations, meticulous data collection, and rigorous statistical analysis. Achieving accurate and reliable results is paramount in research. Therefore researchers strive to design experiments and studies that can isolate and scrutinize the specific variables which they aim to investigate. However, some hidden factors can obscure the true relationships between variables and lead to erroneous conclusions. These covert culprits are known as confounding variables, which in their elusive nature, has the potential to skew results and hinder the quest for truth.
What Are Confounding Variables
Confounding variables, also referred to as confounders or lurking variables, are the variables that affect the cause and outcome of a study. However, they are not the variables of primary interest. They serve as an unmeasured third variable that acts as an extraneous factor. Furthermore, it interferes with the interpretation of the relationship between the independent and dependent variables within a study. Confounding variables in statistics can be categorical, ordinal, or continuous. Some common types of confounding include Selection bias, Information bias, Time-related confounding, Age-related confounding etc.
Additionally, in the world of scientific inquiry, the term “confounding bias”, is used to describe a systematic error or distortion in research findings that occurs when a confounder is not properly accounted for in a study. This can lead to misleading conclusions about the relationship between the independent variable(s) and the dependent variable, potentially introducing bias into the study’s results.
Key Characteristics of Confounding Variables
Key characteristics of confounding variables or confounding factors include:
Confounding factors can distort the relationship between independent and dependent variables in research. Thus, recognizing, controlling, and addressing them is essential to ensure the accuracy and validity of study findings.
Effects of Confounding Variables
Confounding variables play a crucial role in the internal validity of a research. Understanding its effects is necessary for producing credible, applicable, and ethically sound research.
Here are some impacts of confounding variables in research.
1. Lack of Attribution of Cause and Effect
- Confounding variables can lead researchers to erroneously attribute causation where there is none.
- This happens when a confounder is mistaken for the independent variable, causing researchers to believe that a relationship exists between variables even when it does not.
2. Overestimate or Underestimate Effects
- Confounding variables can distort the magnitude and direction of relationships between variables.
- Additionally, they can either inflate or diminish it, leading to inaccurate assessments of the true impact.
- Furthermore, they can also hide genuine associations between variables.
3. Distort Results
- Confounding variables can create false associations between variables.
- In these cases, the observed relationship is driven by the confounder rather than any meaningful connection between the independent and dependent variables.
- This distorts the relationship between the variables of interest, leading to incorrect conclusions.
4. Reduce Precision and Reliability
- Confounding variables can introduce noise and variability in the data.
- This can make it challenging to detect genuine effects or differences.
- Furthermore, the results of a study may not generalize well to other populations or contexts as the impact of the confounders might be specific to the study sample or conditions.
5. Introduce Bias
- If confounding variables are not properly addressed, the conclusions drawn from a study can be biased.
- These biased conclusions can have real-world implications, especially in fields like medicine, public policy, and social sciences.
- Studies plagued by confounding variables have reduced internal validity, which can hinder scientific progress and the development of effective interventions
6. Introduce Ethical Implications
- In certain cases, failing to control for confounding variables can have ethical implications.
- For instance, if a study erroneously concludes that a particular group is more prone to a disease due to a confounder, it may lead to stigmatization or discrimination.
Researchers must identify these variables and employ rigorous methods to account them for ensuring that their findings accurately reflect the relationships they intend to study.
Why to Account Confounding Variables
Accounting confounding variables is crucial in research as it helps the researchers to obtain more accurate results with a broader application. Furthermore, controlling confounders helps maintain internal validity and establishes causal relationships between variables.
Accounting confounding variables also provide a proper guidance to health interventions or policies and demonstrates scientific rigor and a commitment to producing high-quality, unbiased research. Also, researchers have an ethical responsibility to accurately report and interpret their findings.
Researchers must recognize the potential impact of confounders and take adequate steps to identify and measure them to control for their effects to ensure the integrity and reliability of their research findings.
How to Identify Confounding Variables
Recognizing confounding variables is a crucial step in research. Researchers can employ various strategies to identify potential confounders.
Strategies to Control Confounding Variables
Controlling confounding variables can help researchers to establish a more robust research and employing appropriate strategies to mitigate them is necessary in establishing reliability and accuracy in research reporting.
1. Randomization
Randomly assigning subjects to experimental and control groups can help distribute confounding variables evenly, reducing their impact.
2. Matching
Matching subjects based on key characteristics can minimize the influence of confounding variables. For example, in a drug trial, matching participants by age, gender, and baseline health status can help control for these factors.
3. Statistical Control
Advanced statistical techniques like multiple regression analysis can help account for the influence of known confounding variables in data analysis.
4. Conduct Sensitivity Analysis
Researchers should test the robustness of their findings by conducting sensitivity analyses, systematically varying assumptions about confounding variables to assess their impact on results.
Although these measures can control confounding variables effectively, addressing them ethically is crucial in maintain the research integrity.
Examples of Confounding Variables
Here are some examples of confounding variables:
1. Smoking and Lung Cancer:
In a study investigating the link between smoking and lung cancer, age can be a confounding variable. Older individuals are more likely to both smoke and develop lung cancer. Therefore, if age is not controlled for in the study, it could falsely suggest a stronger association between smoking and lung cancer than actually exists.
2. Education and Income:
Suppose a study is examining the relationship between education level and income, occupation and the years of experience could be a confounding variable because certain jobs pay more. Without considering occupation and experience, the study might incorrectly reach to a conclusion.
3. Coffee Consumption and Heart Disease:
When studying the relationship between coffee consumption and heart disease, exercise and habits can be a confounding variable. Unhealthy behaviors like smoking, poor diet and lack of physical activity can contribute to heart disease. Failing to control for these factors could erroneously attribute heart disease risk solely to coffee consumption.
Controlling confounding variables through study design or statistical techniques is essential to ensure that research findings accurately represent the relationships being studied.
Statistical Approaches When Reporting And Discussing Confounding Variables
Statistical approaches for reporting and discussing confounding variables are essential to ensure the transparency, rigor, and validity of research findings. Here are some key statistical approaches and strategies to consider when dealing with confounding variables:
1. Descriptive Statistics
- Begin by providing descriptive statistics for the confounding variables.
- This includes measures such as mean, median, standard deviation, and frequency distribution.
- This information helps to understand the characteristics of the confounders in your study.
3. Bivariate Analysis
- Conduct bivariate analyses to examine the unadjusted relationships between the independent variable(s) and the dependent variable, as well as between the independent variable(s) and the confounding variables.
4. Stratification
- Stratify your analysis by levels or categories of the confounding variable.
- This allows you to examine the relationship between the independent variable and the dependent variable within each stratum.
- It can help identify whether the effect of the independent variable varies across different levels of the confounder.
4. Multivariate Analysis
- Use multivariate statistical techniques, such as regression analysis, to control for confounding variables.
- In regression analysis, you can include the confounding variables as covariates in the model.
- This helps to isolate the effect of the independent variable(s) while holding the confounders constant.
5. Interaction Testing
- Investigate potential interactions between the independent variable(s) and the confounding variable.
- Interaction terms in regression models can help determine whether the effect of the independent variable(s) varies based on different levels of the confounder. Interaction tests assess whether the relationship between the independent variable and the dependent variable is modified by the confounder.
6. Model Fit and Goodness of Fit
- Assess the fit of your statistical model. This includes checking for goodness-of-fit statistics and examining diagnostic plots.
- A well-fitting model is important for reliable results.
7. Graphical Representation
- Utilize graphical representations, such as scatter plots, bar charts, or forest plots, to visualize the relationships between variables and the impact of confounding variables on your results.
These statistical approaches help researchers control for confounding variables and provide a comprehensive understanding of the relationships between variables in their studies. Thorough and transparent reporting and discussion of confounding variables in research involve a combination of statistical studies and a strong research design. Reporting these variables ethically is crucial in acknowledging them effectively.
Ethical Considerations While Dealing Confounding Variables
Ethical considerations play a significant role in dealing with confounding variables in research. Addressing confounding variables ethically is essential to ensure that research is conducted with integrity, transparency, and respect for participants and the broader community. Here are some ethical considerations to keep in mind:
1. Disclosure and Transparency
- Researchers are ethically obliged to disclose potential confounding variables, as well as their plans for addressing them, in research proposals, publications, and presentations.
- Moreover, transparent reporting allows readers to assess the study’s validity and the potential impact of confounding.
2. Informed Consent
- When participants are involved in a study, they should be fully informed about the research objectives, procedures, and potential sources of bias, including confounding variables.
- Informed consent should include explanations of how confounders will be addressed and why it is important.
3. Minimizing Harm
- Researchers should take steps to minimize any potential harm to participants that may result from addressing confounding variables.
- This includes ensuring that data collection and analysis procedures do not cause undue distress or discomfort.
4. Fair and Equitable Treatment
- Researchers must ensure that the methods used to control for confounding variables are fair and equitable.
- This means that any adjustments or controls should be applied consistently to all participants or groups in a study to avoid bias or discrimination.
5. Respect for Autonomy
- Ethical research respects the autonomy of participants.
- This includes allowing participants to withdraw from the study at any time if they feel uncomfortable with the research process or have concerns about how confounding variables are being managed.
6. Consider Community Impact
- Consider the broader impact of research on the community.
- Addressing confounding variables can help ensure that research results are accurate and relevant to the community, ultimately contributing to better-informed decisions and policies.
7. Avoiding Misleading Results
- Ethical research avoids producing results that are misleading due to unaddressed confounding variables.
- Misleading results can have serious consequences in fields like medicine and public health, where policies and treatments are based on research findings.
8. Ethical Oversight
- Research involving human participants often requires ethical review and oversight by institutional review boards or ethics committees.
- Researchers should follow the guidance and recommendations of these oversight bodies when dealing with confounding variables.
9. Continual Evaluation
- Ethical research involves ongoing evaluation of the impact of confounding variables and the effectiveness of strategies to control them.
- Additionally, researchers should be prepared to adjust their methods if necessary to maintain ethical standards.
Researchers must uphold these ethical principles to maintain the trust and credibility of their work within the scientific community and society at large.
The quest for knowledge is not solely defined by the variables you aim to study, but also by the diligence with which you address the complexities associated with the confounding variables. This would foster a clearer and more accurate reporting of research which is reliable and sound.
What are your experiences dealing with confounding variables? Share your views and ideas with the community on Enago Academy’s Open Platform and grow your connections with like-minded academics.
Frequently Asked Questions
Confounding bias is a type of bias that occurs when a third variable influences both the independent and dependent variables, leading to erroneous conclusions in research and statistical analysis. It occurs when a third variable (confounding variable) which is not considered in the research design or analysis, is related to both the dependent variable (the outcome of interest) and the independent variable (the factor being studied).
Controlling for confounding variables is a crucial aspect of designing and analyzing research studies. Some methods to control confounding variables are:
1. Randomization
2. Matching
3. Stratification
4. Multivariable Regression Analysis
5. Propensity Score Matching
6. Cohort Studies
7. Restriction
8. Sensitivity Analysis
9. Review Existing Literature
10. Expert Consultation
Some common types of confounding are selection bias, information bias. time-related confounding, age-related confounding, residual confounding, reverse causation etc.
Confounding variables affects the credibility, applicability, and ethical soundness of the study. Their effects include:
1. Lack of Attribution of Cause and Effect
2. Overestimate or Underestimate Effects
3. Distort Results
4. Reduce Precision and Reliability
5. Introduce Bias
6. Introduce Ethical Implications
To produce valid research, researchers must identify and rigorously account for confounding variables, ensuring that their findings accurately reflect the relationships they intend to study.
Identifying confounding variables is a critical step in research design and analysis. Here are some strategies and approaches to help identify potential confounding variables:
1. Literature Review
2. Subject Matter Knowledge
3. Theoretical Framework
4. Pilot Testing
5. Consultation
6. Hypothesis Testing
7. Directed Acyclic Graphs (DAGs)
8. Statistical Software
9. Expert Review
The potential effect of confounders variables should be discussed or shown!
Thank you