Does it Matter When You Analyze Your Research Data?
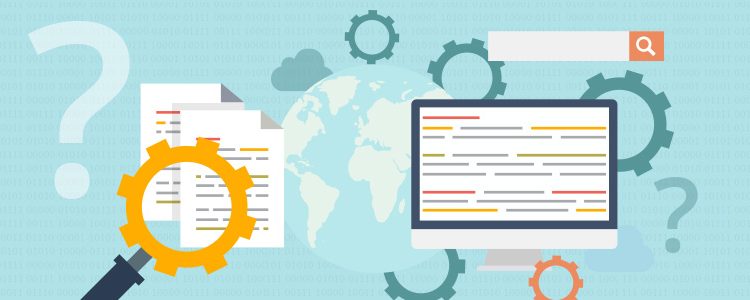
It’s safe to say that there is no research without data. It can be good, bad, or inconclusive, and we pay very close attention to both the methodologies by which it is collected and how human subjects are treated during that collection process. Clinical trials, in particular, utilize data monitoring committees to ensure that every data point is captured in accordance with the established protocol. The perceived quality of research results rests on the statistical analysis of that data, whether the sample was suitably representative, and the extent to which substantive inferences can be drawn from the collected responses and/or test results.
Resource Pressures
Data takes time to collect. Clinical trials are a struggle to fill and surveys often require multiple gentle reminders to reach acceptable participation levels. The parties providing the funding for this research—governments, corporations, and grant agencies—may have unrealistic expectations of the amount of time involved and begin requesting commentary on “early signs” or “initial trends” from the data as it is being collected. When dealing with large sets of previously collected data from other studies, the pressure can be even greater, since there is no perceived justification for delay beyond the processing capacity of the computers and software algorithms working through the data.
Non-Compliant Data
The obvious answer you are forced to give the folks in the suits looking for progress reports is that data doesn’t arrive in sequence. I have found that the coin tossing analogy works well. Just because you hit heads four times in a row, that is no indication of a trend developing, and the probability of getting heads on the fifth toss is still 50/50. In the same manner, five positive responses to a survey or to a test are no indication that the sixth result will be the same. That’s why we place so much attention on randomized data.
The Humanity Factor
For clinical trials that are researching urgent treatments for fatal diagnoses or new strains of a virus such as Ebola, the temptation to respond to the earliest possible signs of a positive result can be overwhelming, especially when dying patients are expressing the earnest willingness to be a trial subject for even the most rudimentary treatment protocol. Biostatistics are obligated to be even more rigorous because of the human lives involved, and when you consider the challenge of responding to an international epidemic like HIV/AIDS, the potential for data loss and corruption when tests are being performed in remote areas on the African continent is enormous.
For data to withstand the scrutiny of rigorous statistical analysis, the integrity of that data can never be in question. Analyzing subsets can be warranted if data comes in from one complete sub-sample while data from others continue to arrive, but the results from that subset can never be inferred or extrapolated beyond what the data truly represents.