Interesting Science vs. Sound Science – All About the Rigor and Transparency Index (RTI)
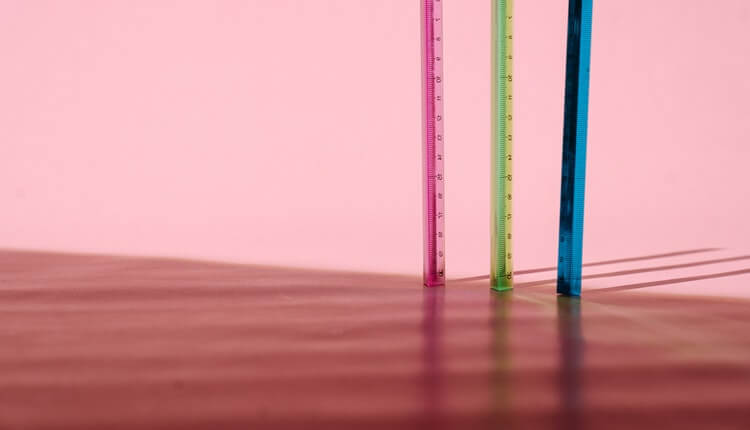
This guest post is drafted by Martijn Roelandse and Anita Bandrowski from SciCrunch. It highlights the unique features offered by SciCrunch to researchers, with a special emphasis on the recently introduced “Rigor and Transparency Index”.
There are certainly interesting scientific stories that we invite people to read about or watch on science television shows. Increasing awareness about the latest scientific advancements is indeed a very good thing to do! However, for most of our lives, we scientists work hard adding pieces to a model believing it is the correct way of how something should work. In either case, we try to discredit the models of other scientists, which we feel might be inaccurate. Honestly, it is far less glamorous doing these activities than simply being an expert and talking about science on a television program. In reality, what scientists do is discuss with colleagues the chances of getting their manuscripts published in journals and how to gain funding for their projects. Certainly becoming famous is possible for scientists, but it is rare. Let us find what SciCrunch has to offer us with its Rigor and Transparency Index tool!
How to Measure the Impact and Rigor of Published Science?
The Journal Impact Factor (JIF) is a measure of how many other papers cited the articles in a particular journal in a year. It is an indicator of how “popular” a journal is amongst scientists. This is a (highly debated) measure of quality, if we consider that other scientists will cite work that is more important and not work that is less important. However, there are some problems with this. Especially if this metric is predominantly used to judge the worth of one researcher over another using a journal metric rather than a metric based on the efforts of an individual person. We now have new metrics for how many times an article appears on social media platforms. This drives sensational science, but not necessarily good careful work.
The worry here is that when a metric emerges people will try to game the metric. They will cite themselves over other scientists, or they will spend precious time on Twitter instead of thinking carefully about new work. There are well-documented examples of abuses in science just to improve these scores (see articles on Scholarly Kitchen, Retraction Watch and Enago Academy).
How to Score Science?
The question here is how can we score the science itself as opposed to counting tweets? Certainly knowing if our studies can be reproduced is one way to do this, however, reproducing a paper is a very long and difficult process. We know from surveys that most scientists have failed to reproduce other scientists’ work, and even their own work (Nature’s survey on reproducibility in research), but there is no way to quantify that. Or is there…?
Around 2010, the US National Institutes of Health led many workshops and authored multiple papers that highlighted the state of our understanding for the kinds of “markers” for reproducibility. Many of these were summarized by the Landis, 2012 study published in Nature. They are now included on the NIH website and have become part of the review process for grants. The core set of reporting standards for a rigorous study design are:
- Randomization: Animals should be assigned randomly to the various experimental groups, and the method of randomization reported.
- Blinding: Allocation concealment: the investigator should be unaware of the group to which the next animal taken from a cage will be allocated.
- Blinded conduct of the experiment.
- Blinded assessment of outcome.
- Sample-size estimation: An appropriate sample size should be computed when the study is being designed and the statistical method of computation reported.
- Data handling: Preregistration rules for stopping data collection should be defined in advance.
- Inclusion and exclusion of data should be established prospectively.
- Replication and pseudo-replication issues need to be considered during study design and analysis.
- An account of sex or other important biological variables
- Authentication of key research resources especially antibodies, cell lines, and transgenic organisms
If we consider these as markers of reproducibility, then we could consider papers that address these markers as generally better. At least we can be more aware of reproducibility issues. It is true that not all of these markers will be present in all studies, and some may simply not be relevant to a study. Nonetheless, in general, studies that use blinding to reduce investigator bias will tend to be somewhat more believable than studies that do not.
Within the EU Horizon 2021 framework programme for research and innovation, researchers will also undergo a review of some of these items for a set of representative papers. While the criteria are not exactly the Landis, 2012 criteria, they generally check for soundness of studies and address some of the same points.
Automated screening tools to the rescue
The field of artificial intelligence has made great strides in detecting relevant patterns in large sets, especially of text. The field has used more and more sophisticated methods to improve pattern detection including adding semantic components of the word, word phrases, and contextual information about the sentence such as parts of speech. Although still far from perfect, currently, these methods have become sufficiently reliable for use in many applications, including detecting sentence constructs of certain types. Things like detection of the sex of experimental subjects is a relatively straightforward example. There are ~20 terms associated with sex and gender that can be “found” in an article including “male” and “female”. These words signal that sex is being addressed, so if the text of the manuscript is processed, then the presence of these terms can be counted (present or absent).
Randomization of subjects into groups or cell line authentication are perhaps a little more complex to understand and find common sentence patterns for, but even these kinds of items can be found using artificial intelligence with reasonable accuracy, and therefore they can be counted.
SciScore – The Amazing Tool
We, at SciCrunch, built a tool called SciScore (text analysis effort was led by Dr. Ozyurt) that counts the number of these criteria that are present and absent in a manuscript and each manuscript is assigned a number that is roughly equivalent to the number of criteria that were found to the number that was expected. Some criteria depend on the presence of other items, for example, cell line authentication is scored only if cell lines are detected. The scoring is a little more complicated than that because some criteria are grouped together, while others like oligonucleotides are detected but not scored. Essentially, a 5/10 means that about half of the things that the tool expected to find were found.
This tool was intended to help authors get their manuscript ready for publication by detecting things that they may have missed, which may be required by journals. For this kind of use, there is a website sciscore.com and a way to use the tool for free, by logging in via ORCID.
Publishers are beginning to use the tool to improve their manuscripts as well. The American Association for Cancer Research allows all authors that submit their manuscripts to work with the SciScore tool before or during review to make improvements to the manuscript before publication. The British Journal of Pharmacology runs the tool as a final check on the manuscripts that are about to be accepted, just to check that all antibodies have the proper RRID identifiers and that none of the cell lines are contaminated. Authors have the ability to still work with their text to make it better before they stake their scientific reputation on the work.
Assessing rigor and reproducibility of 1.6 million papers
This one-by-one author service was the intent of the tool, but SciScore is a very powerful tool that when optimized can check a paper in 1-2 seconds. This is important because there are a lot of papers in science. Last year, we ran an optimized version of the tool on the ~2 million papers in the open access literature (the section that is licensed to allow for text mining). Approximately 1.6 million papers were positively scored. The others were papers that either did not contain an accessible methods section or were outside of the scope, for example x-ray crystallography studies.
The processing time for this job was just over 6 weeks of server time. In a normal setting, the task would have taken a human 15.5 years. This means working 24 hours per day, 7 days per week, with no weekends, and no breaks!
From this massive count of markers of reproducibility emerged a set of data. We looked at the overall numbers by year and by journal to compare this new metric to the Journal Impact Factor. The average score for all papers in a given journal in a given year – The Rigor and Transparency Index or RTI – was then made available at the sciscore.com/RTI website and also as supplemental data to our paper.
The data shows that overall papers address just under 50% of the items that are expected to be addressed, but the percentage is getting better over time. We can see how certain journals are doing, especially those that put a lot of effort into editorial checklists. Nature’s editorial checklist resulted in a change in score for the journal by two points, from the lower end of journals to the high end.
Some journals clearly did better, and many of those were more clinical. We hypothesize that this is because clinical journals already enforce the CONSORT guidelines quite forcefully, and these guidelines include the criteria given by Landis. Some of the basic science journals like the British Journal of Pharmacology and Neuron seem to be the highest scoring from the basic science side. Both journals strictly enforce guidelines. Some journals enforce fewer guidelines, because they are less relevant to their field. So it is probably best to not look at the score as an absolute number. Consider it as a number that might be more useful when looking at a handful of journals that publish similar types of papers (e.g., clinical papers, animal studies, or microbiology studies).
The Rigor and Transparency Index score is not correlated with the Impact Factor, whether we consider it as a percentage in quartiles or raw scores. This is probably not surprising, but kind of interesting.
So how can SciScore and the Rigor and Transparency Index be used?
SciScore can find some of the Landis, 2012 items that were omitted in a manuscript methods section, or if those are written in a way that is not common. It can suggest solutions for those. One solution is to assert the negative “investigators were not blinded during the conduct of the experiment because…”, which is a marker of transparency and should improve the score. Of course, it is generally better, if possible, to address these metrics directly. For example, study data could be coded into group 1 and group 2. Then it could be given for re-analysis to a lab member or a colleague, who is blinded to the control group. This gives additional credibility to the analysis and makes a better study.
For simple omissions like not remembering to put in sex of animals or cell lines, the information can be looked up in the stock center or the animal facility and added. Other simple things to add include identifiers for antibodies, cell lines, and organisms. These identifiers, RRIDs, help to ensure that no mistakes were made in the catalog number in laboratory records. RRIDs ensure that the information about a reagent exists even if the company no longer offers the product or ceases to exist. Software tools can be cited by RRID helping to give credit to colleagues that build these tools.
Things like randomization or preregistration of the study, will not be able to be done at the end of a study. However, they certainly can be considered for the next study to improve the practices in the laboratory.
While this is certainly not yet a common occurrence, the Luxembourg Centre for Systems Biomedicine has partnered with SciScore to help their researchers improve manuscripts before they publish. Currently, they are gathering data from published papers to determine how researchers are faring. Workshops teaching rigor and how to address it, are able to be assessed by comparing participant’s scores for papers before and after workshops.
The Rigor and Transparency Index itself is the average of all scores for a given journal and year. It can be used as a selection criterion for authors who want to be associated with more reproducible journals. Currently, we do not know of any university that is using this index. However, we anticipate a time when such a metric could be used as part of a richer set of metrics about investigators. We certainly hope that making these data easily available will improve its usefulness in making decisions about where to publish.
Have you explored the Rigor and Transparency Index for your articles yet? How do you ensure that your articles have high rigor? Let us know your thoughts in the comments section. If you have any questions related to assessing the impact and quality of your articles, post them here and our experts will be happy to answer them! You can also visit our Q&A forum for frequently asked questions related to research writing and publishing answered by our team that comprises subject-matter experts, eminent researchers, and publication experts.