Strategies for Ensuring Data Quality in Contract Research Studies
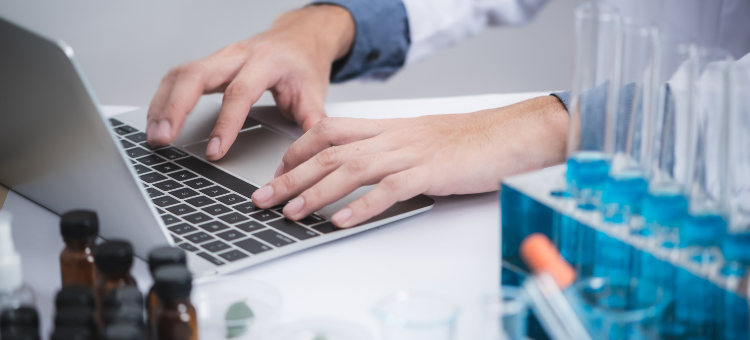
Data quality across the clinical trials will determine the reliability, effectiveness, and speed at which it is taking place. A clinical trial will test the efficacy of the behavioral, medical, or surgical intervention among people. These trials generally take place in four stages, such of which engages more test subjects. Clinical trials directly rely on the data quality to succeed.
Knowledge about following the standard data can help the clinical trial investigator determine whether the method of collecting data is sufficient. If they wish to succeed, a good investigator should aim to enhance data quality across the clinical trials. They should have the know-how about collecting, processing, and interpreting top-notch data rapidly and effectively.
About Clinical Trials Data
Clinical trials performed by a Contract Research Organization (CRO) refer to the information collected during research studies conducted on human subjects to analyze the effectiveness and safety of the latest medical interventions and treatments.
Clinical trials come with a massive range of data points, including medical history, demographics of the patients, treatment results, laboratory test results, and adverse events. The data is essential for advancing medical knowledge and shaping evidence-based healthcare practices.
Importance of Clinical Trials Data
Clinical trial data of the CROs holds a huge essence across the domain of medical research and drug development. It serves as a worthy resource for biostatisticians, researchers, and regulatory authorities that help with the assessment of the treatment’s safety and efficacy. These insights are often drawn out of the clinical trials data that would help in decision-making across different stages of drug development right from the market entry to protocol design.
One of the main beneficiaries of clinical trial data is the biostatistician. They depend on this data to interpret and analyze the outcomes of clinical trials, assess the statistical essence of the treatment impacts, and consider possible adverse effects. They can examine the data to identify patterns, correlations within the data, and trends that allow them to propose the required changes to the upcoming stages of clinical trials to boost the validity and efficacy of the study.
Furthermore, clinical trial data can help in pacing up the availability of new drugs across the market. Regulatory authorities, such as drug regulatory agencies, rely strongly on clinical trial data to assess the latest interventions’ effectiveness and safety. The data would offer important proof for supporting the submission of regulatory applications that enable the authorities to make informed decisions related to drug approvals. Offering reliable and extensive data helps speed up the trials along with the regulatory review procedure to help bring life-saving medications to reach patients.
Strategies to Improve Data Quality In Clinical Trials
The following are the effective ways to boost the data quality prevailing across the clinical trials:
Incorporating Technology Across Data Collection Processes
Try incorporating technology across different stages right from collecting the data to analysis and reporting. In clinical trials, effectiveness and speed are the key. It is possible through technology for collecting and processing data rapidly and accurately. The data collection technology helps enhance the data quality across the clinical trials through:
1. Automating data collection, assessment, and reporting procedures
2. Rectifying errors that occurred during data entry
3. Enhancing speed and accuracy of data entry
Try using tools like EDC or Electronic Data Capture that paces up data collection, customization, and sharing. The abilities of an EDC include the integration of a camera for collecting the image data, data protection, a checklist for the validation of data flow, abilities with data customization, and rest.
Conducting Regular Data Cleaning Exercises
Data cleaning involves fixing or removal of duplicate, incorrect, incomplete, and improperly formatted data within the dataset. It helps with the removal of datasets that should not be present in the database.
One ideal way data gets corrupted is when it arrives from multiple sources and is compiled into one. Data cleaning fixes a couple of issues, including the removal of duplicate or irrelevant observations and fixing structural flaws like naming conventions, typos, and mislabeled categories that hardly fit into the data that needs analysis.
Incorrect data leads to unreliable results, so data-cleaning exercises must be constantly conducted to enhance the quality of data across clinical trials.
Introducing Quality Control Into the Methods of Data Collection
Quality control involves applying methods to investigate whether the data collected across the clinical trials meets the quality standards, goals, and criteria set. You should check a couple of things during quality control, including errors during data transfer from one source to the next, missing details, and duplicate items.
The quality control inspectors will identify and fix the errors earlier on, thereby stopping them from affecting the process of data analysis. The appropriate quality control inspections even ensure that the data stays clean from the collection to the analysis stage, making them highly reliable and practical.
Develop A Data Management Plan
The basic way to boost the quality of data across clinical trials is to implement a robust data management strategy. The DMP or data management plan is a written document with a thorough description of the data you are expecting to get during the clinical trial process.
The data management plan comprises the data on how you would describe, manage, assess, store, and preserve the collected data. The data management plan can help minimize errors while ensuring consistency in the data you collected out of the subjects of your clinical trials. It can also help in the identification and rectifying the errors, enhancing the dependability and accuracy of the data.
Train Your Staff on Data Handling Processes
Data collection involves collaborative efforts. To enhance the data quality across the clinical trials, you should involve the relevant stakeholders to set up the standard operating processes governing data collection. The apportioning of responsibilities and roles allows the seamless flow of the processes involving data collection, leading to quality data collection with minimized errors.
Staff training is also a necessity that allows everyone to use the technologies deployed for data collection. You should create an extensive training plan to ensure individuals handle the data used in clinical trials.
Parting Thought
Data quality is never negotiable whenever it arrives at the clinical trials. The quality of the data collected directly affects the dependency of the trial outcomes. Using unreliable data has a notable impact on the health of the subjects of the clinical trial. Therefore, it is advised to get the process of data collection right at the initial time if you are executing a successful clinical trial.