What is Generative AI’s Role in Financial Fraud Detection?
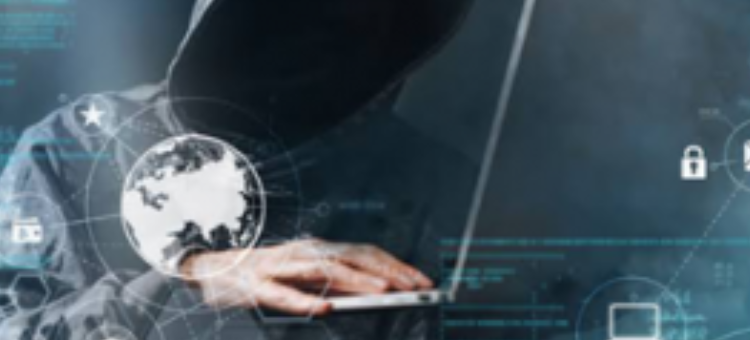
In a world where fraudsters steal a massive $485.6 billion annually, finding effective solutions is crucial. Generative AI provides a promising answer, serving not just as a defense but also as an advanced tool against these evolving threats. By mimicking the intricacies of modern fraud, it helps our financial systems predict and prevent fraud like never before. Want to learn how this technology is transforming fraud detection? Dive into our detailed blog post to discover more!
What is Generative AI?
Generative AI is a subset of artificial intelligence. It is designed to create new content such as text, images, videos, and other data forms. It operates by learning the patterns and structures of input data through advanced machine learning models and then generating new, similar yet original data outputs. This AI capability is particularly distinguished by its use of models like neural networks, which adapt and respond to the input data without explicit programming for specific outcomes.
Key Technologies Behind Generative AI
1. Generative Adversarial Networks (GANs):
Introduced in 2014, GANs consist of two parts: a generator that creates images and a discriminator that evaluates them. This setup helps refine the outputs to be increasingly realistic, as the generator learns to produce data that can fool the discriminator into thinking it’s genuine.
2. Transformers:
Since their introduction in 2017, transformers have become a cornerstone for modern AI. It allows the model to handle and generate large sequences of data, such as text, based on relationships within the data. They are notably used in large language models like the GPT (Generative Pre-trained Transformer) series, which can generate coherent and contextually relevant text on a vast scale.
Comparison with Traditional AI Models
Traditional AI models, like those in supervised learning, need explicit instructions and labeled data to perform specific tasks such as classification. However, generative AI models, including those using self-supervised learning like the GPT series, often work without labeled data. They learn from the patterns in vast amounts of unstructured data, allowing them to predict or generate outputs. This flexibility enables generative AI to tackle a broad range of tasks from creating art to simulating real scenarios without needing detailed programming for each new situation.
Generative AI’s ability to produce human-like content has wide-ranging applications in various sectors, including finance. Here, it can simulate fraudulent activities to help train detection systems, marking a significant advancement over traditional models that are generally limited to narrowly defined tasks.
Types of Financial Fraud
Financial fraud manifests in various forms, each exploiting different aspects of the financial systems:
1. Business Email Compromise (BEC):
This scam involves fraudsters pretending to be company executives or partners to mislead employees into transferring funds or sensitive information.
2. Synthetic Identity Fraud:
Here, criminals combine real and fake information to create new identities, used to open fraudulent accounts or make purchases.
3. Account Takeover:
This involves gaining unauthorized access to a person’s financial accounts and conducting unauthorized transactions.
4. Payment Fraud:
It includes a wide array of deceptive practices like chargeback fraud, advanced fee fraud, and new account fraud, among others.
5. Internal Fraud:
Also known as occupational fraud, this occurs when employees, managers, or executives commit fraud against their employers.
6. Vendor Fraud:
Involves submitting false invoices or impersonating a vendor to divert payments to fraudulent accounts.
Statistical Overview of Financial Fraud Impacts
Financial fraud has substantial economic impacts. In 2024, significant financial losses are continuing to accrue globally due to fraud. For instance, the U.S. alone has seen business and consumer fraud tallying billions annually, with individual and corporate victims spanning across various sectors. These incidents not only result in direct financial loss but also harm reputational integrity and compliance status with financial regulations.
Current Challenges in Detecting Financial Fraud
The detection of financial fraud poses several challenges, primarily due to the sophistication and variability of fraud tactics, which continually evolve. The use of technology, including generative AI, has led to more complex fraud schemes such as deepfake videos and advanced phishing attacks. Next, institutions face significant hurdles due to data silos that hinder the comprehensive visibility of fraudulent activities across different systems and platforms. Regulatory frameworks like the Economic Crime and Corporate Transparency Bill aim to enhance data sharing across institutions, which could improve detection capabilities in the future.
How Generative AI is Used to Simulate Fraudulent Activities to Train Detection Systems
Generative AI significantly enhances fraud detection capabilities by creating synthetic datasets that mirror real transactional behaviors. These datasets are used to train machine learning models, allowing them to learn and recognize patterns of fraudulent activities without compromising the security of real data. This approach improves the models’ ability to detect complex fraud schemes and prepares them to deal with new and evolving types of fraud that have not yet been encountered in the wild. By simulating various fraud scenarios, generative AI provides a robust platform for developing more effective detection systems that can adapt to the dynamic nature of financial fraud.
Case Studies of Financial Institutions Using Generative AI for Fraud Detection
Financial giants like PayPal and American Express are leveraging generative AI to bolster their fraud detection systems. PayPal, for instance, has utilized generative AI and machine learning to nearly halve its loss rate from fraud, even as its payment volumes significantly increased. This success is attributed to generative AI’s ability to rapidly adapt to new fraud patterns and effectively protect customer transactions. Similarly, other financial institutions are using AI-driven strategies to uncover complex activities like money laundering, which often involves subtle and carefully planned transactions that traditional methods may not easily detect.
Benefits of Using Generative AI Over Traditional Methods
Generative AI offers several advantages over traditional fraud detection methods:
-
Real-Time Detection:
It can process vast amounts of data in real time, allowing for the immediate identification of suspicious activities, which is crucial in preventing potential fraud before it causes financial damage.
-
Adaptive Learning:
Unlike static, rule-based systems, generative AI learns from the data it processes, continually improving its ability to recognize and adapt to new fraud tactics as it develops.
-
Data Augmentation:
It generates synthetic data that enhances the training datasets, making machine learning models more robust and less likely to miss subtle patterns of fraud.
-
Reduced False Positives:
By understanding the nuances of transaction data better, generative AI reduces the occurrence of false positives. It can save resources and reduce the inconvenience to customers.
Future Prospects
Innovations on the Horizon for Generative AI in Fraud Detection
Fraud detection is poised for transformation with advancements in Generative AI. Innovations such as AI Risk Decisioning are emerging, combining Generative AI with traditional machine learning to enhance the precision and speed of online transaction security. This includes creating a comprehensive “knowledge fabric” that integrates various data sources for a more nuanced fraud detection process.
Potential Integration with Other Technologies
Generative AI is also set to integrate with technologies like blockchain and the Internet of Things (IoT). Blockchain could enhance the transparency and traceability of data processed by AI, adding an extra layer of security and verifiability. Similarly, IoT devices could provide real-time data that aids Generative AI in detecting fraud more dynamically across various networks and devices.
Expert Predictions for the Role of AI in Future Financial Security Frameworks
Experts predict a significant role for Generative AI in shaping future financial security frameworks. They foresee a continuous evolution where Generative AI not only detects but also predicts and prevents fraud by analyzing patterns and anomalies in vast datasets, thereby staying ahead of fraudsters.
Final Words
Generative AI has already begun reshaping the field of financial fraud detection by enhancing the ability to detect, predict, and simulate fraudulent activities. Its integration into financial security frameworks promises a future where financial transactions can be secure and efficient.
But, the balance between technological advancement and ethical considerations remains crucial. As Generative AI continues to evolve, so does the need for rigorous ethical standards and transparency to ensure that these technologies are used responsibly and do not infringe on privacy or lead to new forms of digital vulnerability.
The financial industry must continue to invest in research and adapt to the advancements in Generative AI App Development technologies. By staying informed and prepared, businesses can leverage these innovations to enhance their fraud detection capabilities while addressing ethical and regulatory challenges.
The dynamic and evolving capabilities of Generative AI App Development services signify a promising future in fraud detection and prevention, underlining the importance of continuous innovation and vigilance in its application.