How to Build a Career in Data Analytics With Generative AI Expertise
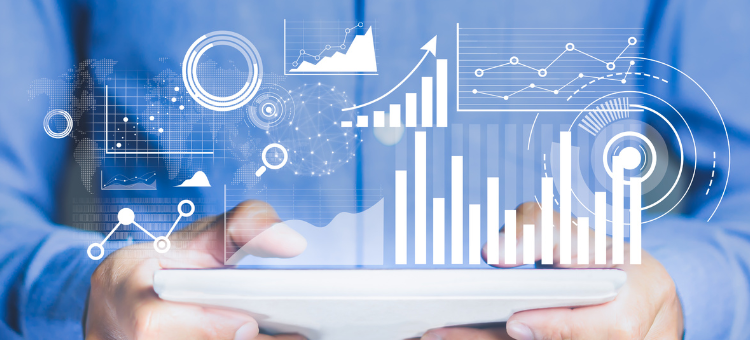
The field of data analytics continues to evolve rapidly, with Generative AI emerging as a game-changing technology. Specializing in Generative AI can significantly elevate your data analytics career, opening up new opportunities and enhancing your skillset. Here’s a comprehensive look at how you can leverage this cutting-edge technology to advance your professional journey:
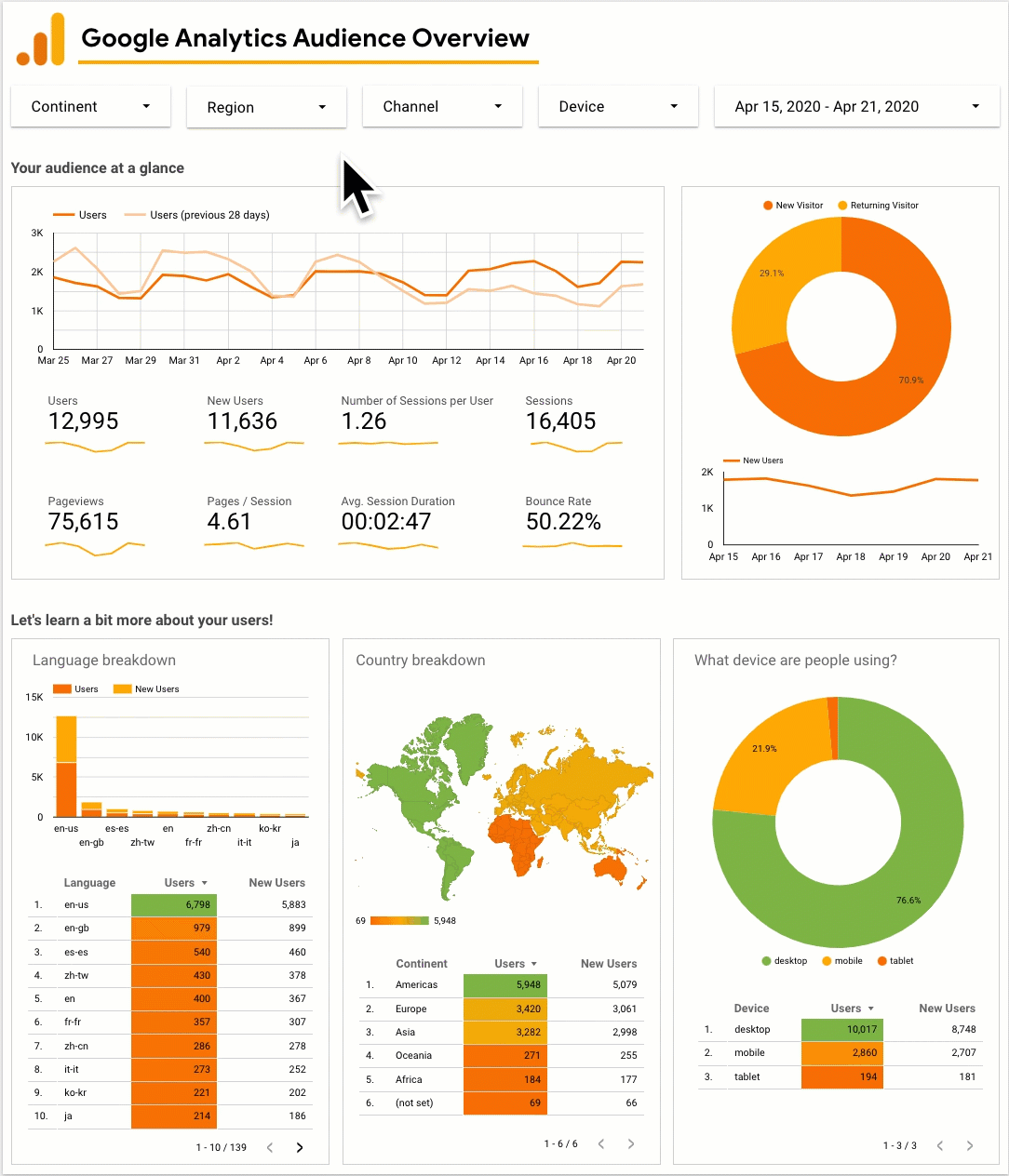
Understanding Generative AI in Data Analytics
Generative AI refers to artificial intelligence systems that can create new content, including text, images, code, and even data. In the context of data analytics, Generative AI is revolutionizing how we approach:
- Data augmentation and synthesis
- Predictive modeling
- Anomaly detection
- Natural language processing and generation
- Automated report writing and insights generation
Benefits of Generative AI Specialization
1. Enhanced Problem-Solving Capabilities
- Tackle complex data challenges with AI-powered solutions
- Generate synthetic data for testing and model training
- Improve decision-making processes with AI-generated insights
2. Increased Efficiency and Productivity
- Automate repetitive tasks in data preparation and analysis
- Rapidly prototype and iterate on analytical models
- Generate reports and visualizations with minimal manual input
3. Expanded Career Opportunities
- Access roles in AI research and development
- Become a valuable asset in industries adopting AI technologies
- Qualify for higher-paying positions as a specialized data professional
4. Innovation and Creativity
- Develop novel approaches to data analysis and visualization
- Create new products and services based on generative models
- Contribute to cutting-edge research in the field of AI and analytics
Steps to Specialize in Generative AI for Data Analytics
Build a Strong Foundation
- Ensure proficiency in statistics, machine learning, and programming
- Familiarize yourself with deep learning concepts and frameworks
Learn Generative AI Techniques
- Study Generative Adversarial Networks (GANs)
- Explore Variational Autoencoders (VAEs)
- Understand transformer models like GPT and BERT
Gain Practical Experience
- Work on projects implementing Generative AI in data analytics
- Participate in Kaggle competitions focused on generative models
- Contribute to open-source Generative AI projects
Stay Updated with Latest Developments
- Follow research papers from leading AI conferences (NeurIPS, ICML, etc.)
- Attend webinars and workshops on Generative AI applications
- Join online communities and forums dedicated to AI and data science
Obtain Relevant Certifications
- Complete courses on platforms like Coursera, edX, or Udacity
- Pursue specialized certifications from tech giants like Google or IBM
- Consider advanced degrees in AI or data science with a focus on generative models
Real-World Applications to Explore
- Financial Services: Risk modeling, fraud detection, and personalized financial advice
- Healthcare: Drug discovery, medical image synthesis, and patient data analysis
- Marketing: Content creation, customer segmentation, and personalized recommendations
- Manufacturing: Quality control, predictive maintenance, and process optimization
- Retail: Demand forecasting, inventory management, and customer behavior analysis
Challenges and Ethical Considerations
As you specialize in Generative AI, be mindful of:
- Data privacy and security concerns
- Ethical implications of AI-generated content
- Potential biases in generative models
- The need for explainable AI in critical decision-making processes
Conclusion
Specializing in Generative AI can significantly elevate your data analytics career, positioning you at the forefront of technological innovation. By developing expertise in this rapidly growing field, you’ll not only enhance your analytical capabilities but also become a valuable asset to organizations looking to leverage AI for data-driven insights and decision-making. Embrace this opportunity to shape the future of data analytics and AI, and watch your career soar to new heights.
5 FAQs on Generative AI Specialization for Data Analytics Careers
1. What prerequisites are needed to specialize in Generative AI for data analytics?
To specialize in Generative AI for data analytics, you should have:
- Strong foundation in statistics and probability
- Proficiency in programming, particularly Python
- Understanding of machine learning algorithms and concepts
- Familiarity with deep learning frameworks like TensorFlow or PyTorch
- Basic knowledge of neural network architectures
2. How long does it typically take to become proficient in Generative AI for data analytics?
The time to proficiency can vary, but generally:
- 6–12 months for those with a strong background in data science and machine learning
- 1–2 years for those transitioning from traditional data analytics roles
- Continuous learning is essential as the field evolves rapidly
3. What are some of the best resources to learn Generative AI for data analytics?
Top resources include:
- Online courses: Coursera’s Deep Learning Specialization, fast.ai’s Practical Deep Learning for Coders
- Books: “Generative Deep Learning” by David Foster, “GANs in Action” by Jakub Langr and Vladimir Bok
- Research papers: Keep up with publications from conferences like NeurIPS, ICML, and ICLR
- Practical projects: Kaggle competitions, GitHub repositories, and personal projects
4. How can Generative AI skills improve my earning potential in data analytics?
Generative AI skills can boost earning potential by:
- Qualifying you for specialized, high-demand roles in AI research and development
- Enabling you to take on more complex, higher-value projects
- Positioning you as an innovator and thought leader in your organization
- Opening doors to industries investing heavily in AI, such as tech, finance, and healthcare
5. What are the ethical considerations when working with Generative AI in data analytics?
Key ethical considerations include:
- Data privacy and protection, especially when working with sensitive information
- Potential biases in AI-generated content or analysis
- Transparency and explainability of AI-driven decisions
- Responsible use of AI-generated data and insights
- Consideration of the societal impact of AI applications in analytics
Disclaimer: The opinions/views expressed in this article exclusively represent the individual perspectives of the author. While we affirm the value of diverse viewpoints and advocate for the freedom of individual expression, we do not endorse derogatory or offensive comments against any caste, creed, race, or similar distinctions. For any concerns or further information, we invite you to contact us at academy@enago.com
- By clicking here, I state that I have read and understood the terms and conditions mentioned above.