How Is Data-Intensive Research Changing Science?
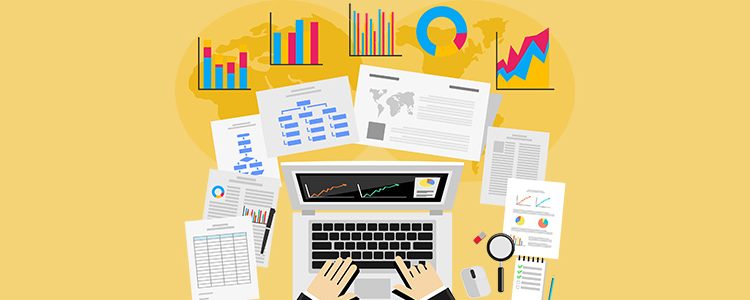
Scientists are particularly interested in collecting data, and the success of each experiment is determined by the novel data that is generated, which can contribute to advancing scientific knowledge. Any scientific research involves making an observation, generating a hypothesis, running an experiment, and collecting data. Traditionally, for any research, the amount of data that was collected by scientists was not very extensive and its analysis did not require the use of technology. Previously, for scientists, technology was used in a very limited manner and assessment of data was not done using algorithms or softwares. However, in the last two decades, significant changes have taken place and various software- and instrumentation-related changes have made both acquisition and analysis of data a very crucial part of research.
Currently, scientists and research are undergoing a paradigm shift. The various developments in statistical softwares, instrumentation, and data-driven science such as computational biology and computational chemistry have led to a new generation of scientists who focus on analysis and interpretation of data that has been obtained. Research projects, such as the Large Hadron Collider, Hubble Telescope, Human Genome Project, are a testament to how science has become extensively dependent on computing and extracting data. Thus, the result of various advances in technology has transformed the field of science. Scientists now have the capacity to conduct high-output experiments, which are essentially data-intensive projects allowing researchers to now collect and store huge amounts of data. Although at first glance, this may seem like a great thing, data-intensive research has some caveats. Also, regardless of these developments, scientists primarily continue to face issues when dealing with large amounts of data. Many research labs do not have scientists who are skilled or trained enough to analyze and interpret such data in a data-intensive research.
Data Analysis: Then and Now
A great example of how data collection has changed is the DNA sequencing technology. In the past, DNA sequencing was somewhat laborious and fairly expensive, forcing researchers to only focus on their gene of interest within their species of interest. This level of analysis and data collection provided sufficient data for a paper to meet the publication requirements. Presently, the cost of sequencing has dropped considerably, thus setting the stage for companies and consortia to have the ability to publish genome sequences. This data has come out at an alarming rate, resulting in the number of publicly available species now standing in the thousands. With these types of publicly available data sets, scientists can now test previous hypotheses against a larger subset of species. However, a biologist is not trained to deal with such vast amounts of data and high-performance computing machines that are needed to store and process these sequences. Moreover, once the data is made available, it would then have to be converted and published in a user-friendly format. Thus, as a result of data-intensive research and its accompanying challenges, many early-stage researchers and graduate students are focusing on improving their knowledge of data science.
Becoming a Data Scientist
Biologists are now using next-generation sequencing tools, physicists are using the Large Hadron Collider and meteorologists are using satellite data collection devices. Nevertheless, in order to work with such data, scientists have to collaborate with various experts to use the wide variety of computational tools and algorithms that are being used today in technology. As scientists have begun to embrace the data revolution, they realize that there are plenty of courses that provide the training they need to gain skills in basic programming, advanced biostatistics, and informatics to name a few.
Some examples computer skills that scientists are using are given below:
- Using Macros – In programs, such as Excel or ImageJ, macros allow you to perform a repeated task. Implementing this tool into data analysis saves a lot of time.
- Data management tools – Programs, such as SQL, R Tool, SPSS or SAS help manage huge amounts of information such as high-throughput sequencing data.
- Coding – Python is the most common coding language that is typically used in data science, along with Java, Perl or C/C++.
New Career
While established scientists are now gaining new skills in order to keep up with the influx of high-throughput data that comes from data intensive research, the notion of gaining these skills to become a data scientist is actually now a full career for some scientists. Many Ph.D. and Master’s programs now offer training specifically in data science. The field of data science continues to be very promising and there is no doubt that scientists will rely on such skills even more in the future.