Microsoft Algorithm FaST-LMM — How Does it Help Researchers?
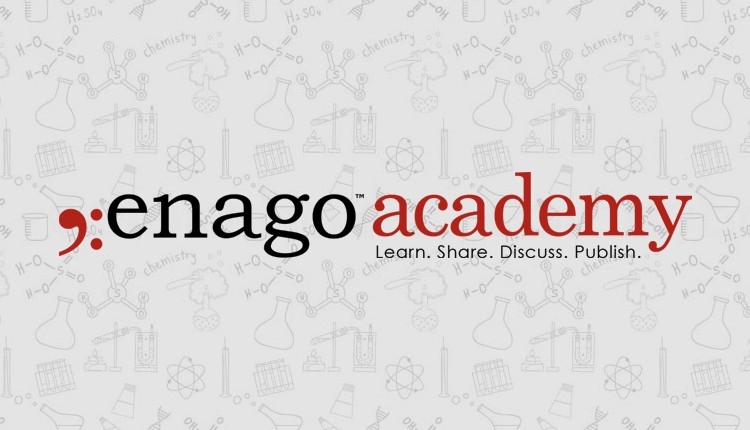
Addressing Data Limitations
Having access to large volumes of big data is usually considered to be a significant advancement in research. Yet, unless that data is coded and stored in a manner that facilitates extraction, the volume issue can actually prove to be a detriment rather than a bonus.
Supercomputers were initially the logical answer, but not any more. The deluge of data has continued to the point where the storage capacity of those computers and the processing speed of their central processing units (CPUs) are starting to restrict both the speed and breadth of data that can be processed.
FaST-LMM: An Alternative Approach
Rather than continuing to build bigger and faster supercomputers, Microsoft Research approached the problem from the perspective of a better algorithm that could process the data faster.
Microsoft algorithm FaST-LMM, which stands for Factored Spectrally Transformed Linear Mixed Models, leverages the physical structure of server farms with thousands of compute nodes and cloud storage, to reduce processing periods from years to days or even hours.
Such reductions may seem unimportant to a researcher looking to access a library database for a literature review, but taking new approaches to how data is deliberately coded for further analysis is a critical step in managing ever large datasets.
Up to now, the emphasis has been on building our capacity to gather, store, and share data, with minimal concern for consistency in how that data was originally stored. New algorithms hold immense promise for how that data can be stored and searched in a more user-friendly and efficient manner.
FaST-LMM Works When Data is Incomprehensibly Big!
The potential for Microsoft algorithm FaST-LMM, or the ones similar to it, becomes apparent when you consider the current use of genome-wide association studies (GWAS) to relate genetics to diseases such as diabetes and different types of cancers.
The studies focus on tiny parts of the human DNA sequence that are highly variable between people, called single-nucleotide polymorphisms (SNPs). Up to 500,000 SNP’s can be compared at a time.
Early expectations were that diseases could be tagged with three or four SNP’s, but studies have found that hundreds could be involved, which has escalated the scale of the datasets considerably.
Playing Catch-Up
The GWAS studies illustrate a larger problem that may continue to burden academic research in general. The $2.7 billion of federal funding that was invested to map the human genome has now reduced the costs of these tests to where they are considered “relatively inexpensive.”
However, that investment hasn’t been matched with similar projects to develop the tools necessary to store, manage, and analyze all the data generated by those tests.
Those resources must be considered in terms of equipment, software, and trained personnel to leverage what scientist compare to a treasure trove of discoveries just waiting to be accessed.