Quick Guide to Biostatistics in Clinical Research: Multiplicity
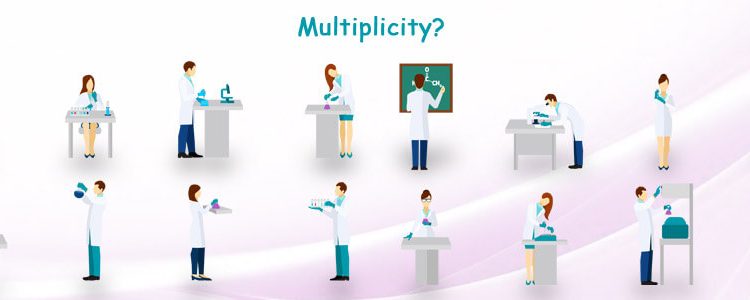
Clinical trials very often involve performing multiple comparisons on the clinical data. This leads to multiplicity problem. The multiplicity problem occurs because the more statistical tests one runs the higher the likelihood that the statistical significance will be achieved. If the level of significance is 0.05, the probability of getting at least one statistically significant result is 64% if 20 tests are done and rises to 99.4% if 100 tests are done. The problem with multiple testing is that it is highly likely to obtain a p-value below the level of significance even when there is no true association. There are guidelines on when statistical corrections are required to deal with the multiplicity problem and when these corrections are not necessary. Clinical trials that are free from this multiplicity issue have following characteristics:
- Only two treatment groups
- Utilize one primary variable
- Have a strategy in place to confirm the results using a single null hypothesis involving the primary variable, which lacks any interim analysis.
Multiple testing occurs when more than one independent statistical tests are performed on the data. This can occur in any of the clinical trial phases. For instance, multiple testing would occur if a medicine in a clinical trial targets more than one symptom. Relief from each symptom would be considered an endpoint. It is, therefore, possible that the drug being tested has a statistically significant effect on patient symptoms just because multiple statistical tests were applied. This problem can also be introduced if there are many subgroups in a clinical trial.
Multiplicity Adjustment
Multiple testing can be done without introducing false associations if certain statistical measures or corrections are applied. The Bonferroni correction (α/n) is one of those measures. The Bonferroni correction adjusts the significance level (α) by dividing the cutoff point (usually 0.05) by the number of independent tests being done (n). This correction is known to be conservative and can lead to high rate of false negatives.
Bioinformatics, especially genome-wide association studies, frequently perform thousands of independent tests on the same data set. In this case, controlling the false discovery rate (FDR) might be the better correction to use. The FDR determines the proportion of false positives (type I error) among all the results deemed significant. The FDR should be less than the level of significance (α). This correction, thus, helps to control the type I errors.
Clinical Trial Adjustments
Another approach that researchers can take when performing medical research or clinical trials is to choose a single primary endpoint. As an example, a cold medicine might have desired endpoints of reducing congestion, lowering fever, and alleviating post-nasal drip. Instead of determining if the drug has a statistically significant effect on all three symptoms, alleviating congestion could be made the primary endpoint for biostatistical calculations.
If any of the clinical trial phases involves multiple subgroups, the statistical tests can still be performed. The level of significance should not be altered after the trial has been completed, the number of subgroups should be kept low, and the results should be biologically plausible and aligned with the external evidence. If these conditions are not met, the results should be viewed as preliminary and another clinical trial should be planned to closely examine other statistically significant results.
The multiplicity curse may be the reason that Phase III clinical trials fail due to many endpoints and comparisons. (Phase III trials are supposed to confirm the safety and efficiency data derived from Phase II trials). This may arise due to many reasons. Repeated measures during the clinical trial can be one of them. This might happen if the study requires measurements of the same patient over a given time period and can be avoided by using a summary measure such as the mean or median of all the readings. One could also reduce the number of time points in the clinical trial. It would be best to use regression analysis if repeated measures are to be taken. We will look at linear and multiple regression in the next article in this series.