Quick Guide to Biostatistics in Clinical Research: Sample Size
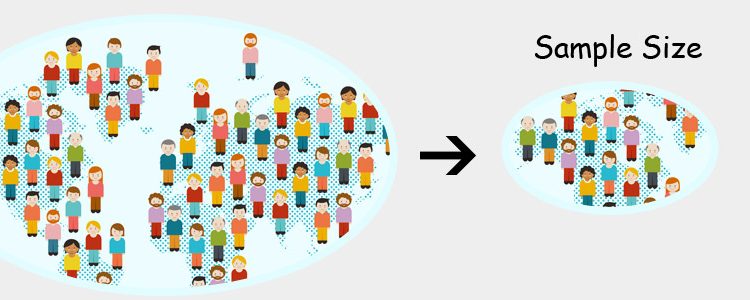
Biostatistics plays a critical role in helping clinical researchers determine the value of their findings. It is important in calculating the sample size, determining the power of a study, and assessing the statistical significance of the results. The p-value is very commonly reported as an outcome of statistical hypothesis tests. As we have seen earlier in this article series, the hypothesis testing depends on the level of significance or α. Usually, α is set at 0.05. If the statistical hypothesis test has an associated p-value, that is less than α, then the relation between an outcome and a predictor variable is considered statistically significant.
Misconceptions with p-value
Though p-value is an important statistical indicator, it has often been misinterpreted. For instance, if p-value is 0.05, that does not mean that the finding is clinically relevant. A finding will have clinical significance only if the endpoint being studied actually has an impact on how a patient would be treated or diagnosed. Sometimes, it is impossible to directly study the variable that is relevant, so another variable or trait has to be used. A result involving this secondary trait may be of limited clinical value. When the finding is statistically significant, but the effect size is small, this may not have a large impact on the problem that you are studying.
Another misconception is that observed data would occur only 5% of the time if the null hypothesis was true. In fact, the p-value is the probability that the observed data, plus more extreme data, would occur if the null hypothesis was true. More extreme data are usually unobserved and complicate the issue. Some people think that the p-value should be written as an inequality. This is based on the p-value serving as a cutoff—the observed data is either statistically significant or not. However, reporting the absolute p-value of the hypothesis test indicates how much evidence is there to reject the null hypothesis. For instance, a p-value between 0.01 and 0.05 could be considered moderate evidence against the null hypothesis being true, whereas a p-value of 0.001 could be considered very strong evidence against the null hypothesis being true.
It is important to remember that a statistically significant result was initially meant to be an indication that an experiment was worth repeating. If the replication studies also yielded statistically significant results, then the association is unlikely due to mere chance. The p-value should not be separated from experimental data or its real world applications.
Sample Size
One of the early steps in designing a clinical study is determining the sample size. If this calculation is not done correctly, it may result in quantitative research that is unable to detect the true relationship between the predictor and outcome variables. The sample should be of appropriate size and representative of the population being studied. Sample size calculations require that the effect size, type I error level, type II error level, and the standard deviation are known.
- The effect size, δ, is the smallest difference that is clinically or biologically meaningful. This is the difference between the means of the two groups being compared. The effect size can be determined from the literature or through a pilot study. A small δ will require a large sample size in order to detect or observe the effect.
- The type I error level, α, is the significance level cutoff. The smaller the α, the larger the sample size.
- The type II error level, β, is the probability of rejecting the null hypothesis when it is true. (1-β) is the ability to detect a difference between the groups being compared when one actually exists. This is also known as the power of a study. As β decreases, the sample size will have to increase in order to maintain the power of the study.
- The standard deviation, σ, represents the variability of the data. Greater the σ, larger the sample size required to attain the required power and level of significance. Sample size calculations can be precision-based or power-based.
The power (1-β) of a clinical trial is one of the parameters that need to be decided beforehand. Power has an impact on sample size. Usually, studies are designed to have at least 80% power. This means that you will have an 80% chance of detecting an association if one exists. This will result in a smaller sample size than the one required for 95% power, implying higher the power, larger the sample size. The 80% threshold represents a compromise between the likelihood to detect an effect, if one exists and the need of an incredibly large sample size. Sample size tables and software programs are available to determine the sample size, however, it can be calculated using mathematical expressions depending on the study design, types of statistical tests used, and value of the parameters discussed above. Attrition or dropout rate should also be factored in sample size calculation. For example, if the dropout rate is expected to be 20%, then the sample size should be increased by a factor of 1/(1-0.2) i.e. by 25%.
In our next article in this series, we will examine the multiplicity problem in clinical trials and what adjustments can be taken into consideration.