“Statistical Significance” – A Term of the Past?
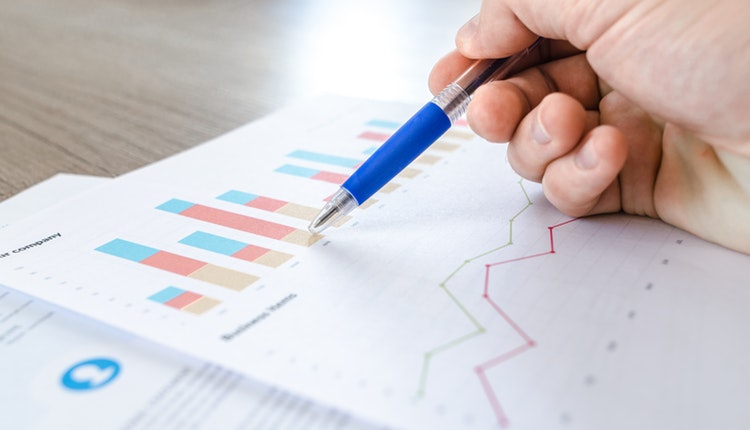
You can also listen to this article as an audio recording.
What do you do when you are trying to decide if your treatment is effective?
In your research study, there are two groups (or more), where one group (or the test group) of subjects receives specific treatment for a condition, and the other group does not. The control group receives a placebo or “blank.” Next, you run your data of the test and the control group through statistical analysis to prove that your treatment is more effective than your control group and that this is decided by p-value and termed “statistically significant.
Not every subject will react the same way to treatment, and one can never be 100% certain of an effect. This is where I come in. I am the ‘p-value,’ I am used to deciding whether a treatment is effective in most cases. Scientists decided that if my value was less than 0.05 (p < 0.05), the results were “statistically significant.” I rose to fame quickly. Scientists all over the world calculated my value. My numbers were cherished and relied upon to make decisions and even policies. I was the gold standard. I was quoted in just about every paper and at every conference.
Don’t use or trust “statistically significant”
Now my glorious reputation is under threat. It turns out I have been overused and manipulated, and my future as a reputable statistical standard is threatened. The American Statistical Association (ASA) has told researchers that they should use me cautiously. They are offering alternative ways to verify differences in treatment groups.
Statisticians claim that calculating statistical significance does not “prove” the null hypothesis. Furthermore, my p-value has resulted in many false conclusions. For example, two studies analyzing the same side effect of an anti-inflammatory drug made two different conclusions. One paper claimed the drugs did not cause atrial fibrillation in patients (p-value = 0.091) and another group found that they did (p value= 0.0003). Both studies were supported by me, the p-value. Cases such as these decrease my credibility. I am supposed to be the magic number that proves an effect is reproducible.
Then there is p-hacking. Researchers have discovered my Achilles heel. They can manipulate the data to shift the p-value towards the result they want. This is especially relevant to large sample groups. The larger the sample size, the more data they collect and the more likely their desired p-value will be achieved.
Post-P-Value Era
Is there room for me in a ‘’post-p-value era’’? Nicole Lazar from the University of Georgia said the time has come to a stop using “statistically significance”. The reproducibility crisis and lack of trust from the public can largely be attributed to scientists misusing me. Unfortunately, there is no magic number or one statistical method that can be used to make decisions about hypotheses.
Can we still quantify the likelihood of a hypothesis?
How can researchers make a decision when sometimes a treatment has an effect and sometimes it does not? A hypothesis is not likely to be proven 100%. I may need to retire (or semi-retire) and share my glory with some of my statistical cousins including “confidence index” or “Bayesian measures.”
Alternatives to “statistically significant”?
There is no straight forward substitute for the words “statistically significant.” The ASA proposes that scientists change the way they communicate their observations by including the probability of an effect occurring. One such statistical calculation is the false positive risk (FPR). This tells your audience the degree of impact of the study has occurred by chance.
Organizations such as the Food and Drug Administration (FDA) need to be careful when they report their research. They must err on the side of caution to avoid legal action.
Have you found a better way to prove your hypothesis? Let us know in the comments section below?