Overcoming Skepticism Through Experimentation: The role of AI in transforming peer review
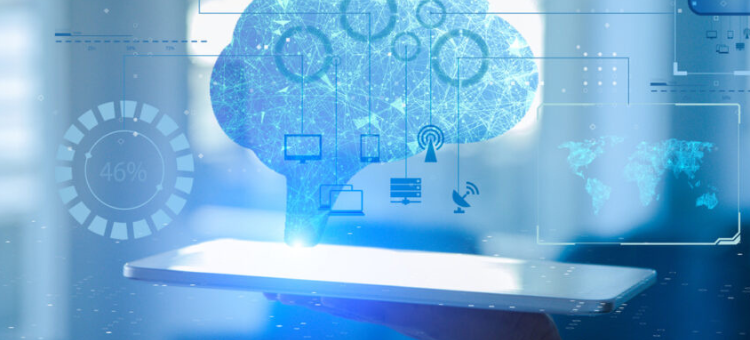
Artificial intelligence (AI) has made significant strides across various domains, yet one critical area within academic publishing remains hesitant: peer review. This foundational system, integral to the advancement of knowledge, lags behind in adopting technological means to improve itself. While concerns about integrating AI into peer review are valid, it is essential to explore how experimentation with AI can address existing challenges and enhance the process.
Challenges in the Current Peer Review System
The traditional peer review process faces several significant problems. One of the most pressing issues is the rising volume of submissions. The exponential increase in research papers overwhelms the capacity of available reviewers, leading to delays in the publication process. Academics, already balancing teaching, research, and administrative duties, often experience reviewer fatigue and time constraints. This additional responsibility can result in delayed feedback or less thorough evaluations. Human bias is another concern affecting the objectivity and fairness of reviews. Unconscious biases related to an author’s institution, nationality, gender, or research area could influence the evaluation process. Moreover, as research becomes increasingly interdisciplinary, finding reviewers with the necessary expertise across multiple fields becomes more challenging, potentially compromising the quality of reviews.
Non-AI Solutions to Peer Review Challenges
To mitigate these challenges, several non-AI strategies have been proposed. Implementing reviewer credits and incentives can motivate timely and thorough reviews. Providing reviewer training programs can improve the quality and consistency of evaluations, helping to reduce biases and enhance assessment skills. Additionally, adopting open peer review practices, where reviewer identities are disclosed, can foster accountability and encourage more constructive feedback. While these solutions offer potential improvements, they may not fully address the scale and complexity of the challenges faced by the peer review system today. The sheer volume of submissions and the increasing complexity of interdisciplinary research necessitate more robust solutions.
Advantages of AI in Peer Review
Integrating AI into the desk and peer review processes presents several compelling advantages. AI can enhance efficiency and speed by rapidly processing large volumes of submissions. It can perform initial screenings for relevance, compliance with guidelines, and detect plagiarism, thereby reducing turnaround times. Furthermore, AI can improve the matching of reviewers and manuscripts by analyzing content to pair submissions with the most suitable reviewers based on expertise, availability, and past performance.
In terms of the actual review process itself, there are countless possibilities. AI is capable of generating structured peer review reports or can be used to ensure that human reviews are thorough. In evaluating interdisciplinary research, AI tools can integrate information across different fields, assisting in assessments that may challenge human reviewers. By identifying statistical errors and methodological flaws, AI can improve the overall quality of published research. AI algorithms can apply consistency in evaluation by using standardized criteria uniformly across all manuscripts, minimizing variability due to human subjectivity.
Concerns Regarding AI in Peer Review
Despite these advantages, there are legitimate concerns about incorporating AI into peer review. Most of the positive results of AI in peer review come from studies in computer science, where the volume of data and a certain comfort with technological solutions create an ideal testing ground. The guarded optimism regarding the potential of AI assistance in peer review is not mirrored in health and biosciences, where the volume of research is arguably much higher. Fields like healthcare, which would benefit most from AI’s ability to quickly and effectively sift through enormous datasets, remain hesitant.
Current AI models have limited data and information at their disposal and hence do not possess the deep understanding required to evaluate complex or highly specialized research, especially in cutting-edge fields. Privacy issues also arise, as using AI involves handling sensitive manuscript data, raising concerns about data security, confidentiality, and compliance with privacy regulations. For instance, the National Institutes of Health (NIH) has prohibited the use of generative AI technologies in the peer review process due to such concerns.
Publisher readiness is another challenge. Implementing AI systems requires significant investment and technical expertise, and not all publishers may be equipped to adopt these technologies effectively. There is also the risk of algorithmic bias, where AI systems could perpetuate existing biases present in their training data, potentially leading to unfair assessments. Additionally, skepticism about AI’s decision-making processes and a lack of transparency could hinder trust among authors, reviewers, and editors.
The Case for Experimentation despite Concerns
Acknowledging these concerns is crucial, but they should not halt the exploration of AI’s potential benefits. Instead, they highlight the need for careful, responsible experimentation. Many potential futures have been envisioned, including a fully AI-driven review process, a human-AI collaborative review with humans focusing on aspects AI cannot handle, an AI check of human reviews, and a human check of AI-generated reviews.
By conducting pilot programs, publishers and academic institutions can gather valuable insights into how AI can be integrated into the peer review process without compromising quality or security. Proposed scenarios include AI adoption at the publisher level, allowing data access for models, and creating AI playgrounds to get feedback from researchers and reviewers. Familiarity breeds trust, and it is through these low-stakes environments that AI can begin to integrate more seamlessly into peer review.
Advancing Through Education and Collaboration
Collaborative development is essential. Working with AI experts, ethicists, and stakeholders can help tailor systems to address specific needs while prioritizing ethical considerations and data security. Positioning AI as a tool to assist rather than replace human reviewers can alleviate fears of over-reliance on automation and maintain the essential human judgment in peer review.
Sharing anonymized peer review data can enhance AI models while maintaining confidentiality. Such cooperation ensures that AI tools evolve in ways that align with the academic community’s values and standards. By pooling data and resources, AI tools could become more adept at addressing the specific needs of different academic fields, particularly in areas where AI skepticism is higher.
AI proponents like Ethan Mollick advocate for reimagining how we consider AI assistance in education and research practices. Likewise, improving AI literacy within academia is vital for overcoming skepticism. Educational programs and workshops can help researchers understand AI’s capabilities and limitations, fostering a more informed and constructive engagement with these technologies.
Conclusion
In conclusion, the challenges facing the peer review system are significant, and while concerns about AI are valid and must be addressed, they should not prevent experimentation with new solutions. By thoughtfully integrating AI into the peer review process, we can tackle issues of efficiency, bias, and the growing volume of research. Experimentation, guided by ethical considerations and collaborative efforts, can transform peer review to better serve the advancement of knowledge. It is not about handing over control to machines but about developing open, transparent AI systems that academia can tailor and refine. The more inclusive and collaborative this process, the more likely it is that AI will fulfill its potential as a valuable tool, not a threat.
This article, “Overcoming Skepticism Through Experimentation: The role of AI in transforming peer review” was published by The Scholarly Kitchen.