Are Synthetic Data a Real Concern? Substantive Predictions and Mindful Considerations Through 2030
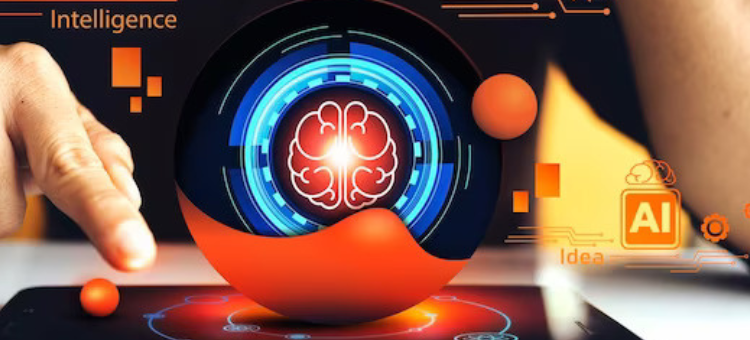
The use of generative AI, exemplified by advanced models like ChatGPT and BARD have catalyzed a revolutionary approach to data generation. Scientists, driven by the quest for innovative solutions and a deeper understanding of complex phenomena, are increasingly turning to these AI tools to craft synthetic datasets. Unlike relying on a training set as a foundation, synthetic data is generated independently, fostering greater diversity and unpredictability in results. This signals a significant change, as we move from depending on natural or experimentally obtained data to also using artificially created datasets. In some cases, these new datasets are not just adding to but replacing traditional methods, and there’s a concern that they might be a result of the AI technology’s ability to generate data that might not be entirely accurate or reliable.
While synthetic data proves invaluable in domains where obtaining real-world data is challenging, expensive, or ethically constrained, there is a growing apprehension about potential threats. The ability of AI technology to generate data independently poses questions about the accuracy and reliability of these artificially created datasets. We stream through the fine line between leveraging the benefits of generative AI for synthetic data and innovative solutions, while ensuring the trustworthiness of the data produced.
Balancing the advantages of diversity and unpredictability with the need for accuracy becomes crucial as synthetic datasets replace traditional methods of research data analysis and collation. Addressing these concerns associated with the outpour of synthetic data generation — is it a necessity particularly invaluable in domains where obtaining real-world data is challenging, expensive, or ethically constrained, or yet another threat to research integrity?
Let’s explore the responsible adoption of synthetic data and predict the future of data-driven research.
Real World Evolution of AI in Hypothesis and Data Generation
AI’s role in hypothesis generation traces back over four decades. In the 1980s, Don Swanson, an information scientist at the University of Chicago, spearheaded literature-based discovery—a ground-breaking text-mining venture aimed at extracting ‘undiscovered public knowledge’ from scientific literature. Swanson’s software, Arrowsmith, identified indirect connections within published papers, successfully proposing hypotheses from vast datasets, like the potential of fish oil to treat Raynaud’s syndrome. Today, AI systems, armed with advanced natural language processing capabilities, construct ‘knowledge graphs’ and identify potential links between various elements, propelling advancements in drug discovery, gene function assignment, and more.
In October 2023, amidst the announcement of the Nobel laureates, a group of researchers, including a previous laureate, met in Stockholm to discuss the expanding role of artificial intelligence (AI) in scientific processes. Led by Hiroaki Kitano, the chief executive of Sony AI and a prominent biologist, the discussions unfolded around the potential creative contributions of AI in scientific endeavors. Notably, Kitano had previously proposed the Nobel Turing Challenge, envisioning highly autonomous AI systems, or ‘AI scientists,’ capable of Nobel-worthy discoveries by 2050.
Synthetic Data and AI Hallucinations: Catalysts for potential medical breakthroughs?
AI’s potential in medicine unfolds through its ability to assist scientists in brainstorming. The unique ability of AI to generate hypotheses based on synthesized data, often likened to ‘hallucinations,’ has the potential to revolutionize the traditional boundaries of medical inquiry.
A review on synthetic data in healthcare explores the role of synthetic data in health care, addressing the controlled access limitations to real data. It identifies seven use cases, including simulation, hypothesis testing, and health IT development. While emphasizing the preference for real data, the review highlights synthetic data’s potential in overcoming access gaps, fostering research, and informing evidence-based policymaking, citing various accessible datasets with varying utility.
1. Unleashing the Creative Spark
Large language models, shaped by extensive exposure to diverse textual data, exhibit a distinctive capability – they can ‘hallucinate’ or generate hypotheses. AI’s ‘hallucinations’ should not be misconstrued as mere whimsical outputs. This process, akin to a creative spark, allows AI to propose potential truths or connections that might elude human researchers.
By presenting hypotheses that appear plausible within the vast landscape of medical knowledge, AI aids researchers in identifying promising leads, prompting further investigation into uncharted territories.
2. Emphasis on ‘Alien’ Hypotheses
Sociologist James Evans, a proponent of AI’s potential in drug discovery and other medical breakthroughs, underscores the significance of AI in producing ‘alien’ hypotheses — ideas that human researchers might be unlikely to conceive. The essence lies in AI’s capacity to explore unconventional paths, connecting disparate elements within vast datasets that may not be immediately evident to human researchers.
3. Beyond Human Cognitive Constraints
The human mind, while immensely powerful, operates within certain cognitive constraints. AI, free from these limitations, explores avenues that might seem alien or unconventional to human thinkers. This escape from the expected norms becomes a catalyst for disruptive thinking, potentially leading to novel approaches, treatments, or diagnostic methods in the medical domain.
4. Enhancing Research Agility
In a field where adaptability and agility are paramount, AI’s contribution to medical research lies in its ability to suggest hypotheses that might align with emerging trends, recent findings, or unconventional patterns. This accelerates the pace of discovery, enabling researchers to navigate complex medical landscapes with greater efficiency.
Predicting the Future: AI-generated synthetic data through 2030
Steering into the future, we predict that AI-generated hypotheses or synthetic data stands on the brink of transformative evolution, with several noteworthy predictions shaping the landscape of scientific research.
As per a Gartner report, the dominance of synthetic data over real data in AI models is anticipated to be prevalent by 2030.
Source: Gartner
1. Enhanced Privacy-Preserving Research
As privacy concerns continue to shape data usage policies, synthetic data generation methods will play a pivotal role in facilitating research that involves sensitive information. For example, in healthcare, where patient privacy is paramount, researchers can replace actual patient information with synthetic data.
This approach will contribute to the ethical advancement of medical research while adhering to stringent data protection regulations.
2. Addressing Data Scarcity in Specialized Fields
Synthetic data is expected to become a cornerstone in addressing data scarcity issues, particularly in highly specialized research areas, such as astrophysics, where observational data is limited, will increasingly rely on synthetic datasets to train machine learning models for tasks like galaxy classification and exoplanet detection. This trend will foster innovation by providing researchers with more extensive and diverse datasets for analysis.
3. Advancements in Materials Science Research
In the field of materials science, where experimental data can be both expensive and time-consuming to generate, synthetic data will emerge as a valuable asset. Researchers will use advanced generative models, such as Large Language Models (LLMs) and Generative Adversarial Networks (GANs), to simulate and predict material properties. This application will expedite materials discovery and contribute to the development of novel technologies.
4. Improving Reproducibility and Rigor
We anticipate that synthetic data will play a crucial role in enhancing the reproducibility and rigor of research studies. By providing researchers with access to diverse datasets that simulate real-world scenarios, synthetic data generation methods will contribute to the robustness of findings.
5. Ethical Considerations and Bias Mitigation
As the use of synthetic data becomes more prevalent, researchers and publishers will need to grapple with ethical considerations and potential biases introduced by generative models. Future scholarly publishing standards may include guidelines on the responsible use of AI, including synthetic data, ensuring transparency in methodologies and addressing any unintended biases that could impact research outcomes.
Furthermore, researchers and publishers may (definitely, should!) collaborate to establish standardized validation and benchmarking protocols for studies using synthetic data. This will involve defining criteria for assessing the quality and reliability of synthetic datasets, ensuring that results derived from these datasets are comparable and trustworthy across different research endeavors.
6. Integration With Simulation-Based Research
The integration of synthetic data generation with physical simulations will become more sophisticated. This convergence will be particularly evident in fields like autonomous vehicles, where realistic training scenarios are essential. Researchers will increasingly use synthetic data to complement physical simulations, ensuring that machine learning models are well-prepared for diverse and rare real-world events.
For text, synthetic data generation plays a crucial role in various tasks beyond summarization and paraphrasing of research articles and references used during a study. It can be employed for tasks such as text augmentation, sentiment analysis, and language translation. By exposing the model to diverse examples and variations, synthetic data helps improve the robustness and adaptability of natural language processing algorithms. Furthermore, generating images using synthetic data can offer increased diversity by incorporating learned characteristics.
A Future Much Better Aligned!
With the future being AI, these predictions not only aim to reshape the landscape of scientific inquiry but also navigate ethical considerations with a heightened sense of responsibility. The collaborative efforts of researchers, ethicists, and technologists will steer this evolution, unlocking the full potential of AI for ground-breaking discoveries and advancements across diverse fields of knowledge.
After the global synthetic data generation market reached USD 123.3 million in 2021, it is anticipated to achieve a significant compound annual growth rate (CAGR) of 34.8% till 2030.
By 2030, the convergence of AI and research expects a future where ethical considerations stand at the forefront of scientific discovery, ensuring a practical coexistence between technological progress and research integrity. However, challenges persist. AI systems often rely on machine learning, demanding vast datasets. The research community envisions the need for AI that not only identifies patterns, but also reasons about the physical world.
These predictions could be a reality — with each stride in AI’s creative role moving us closer to a new era of scientific discovery.
Be a part of the solution: Post your approaches, strategies, or challenges in maintaining research integrity with AI on our open platform. Your insights may inspire others and shape the future of research and publishing.
Comments are closed.