Will Changing the P-Value Fix Reproducibility in Science?
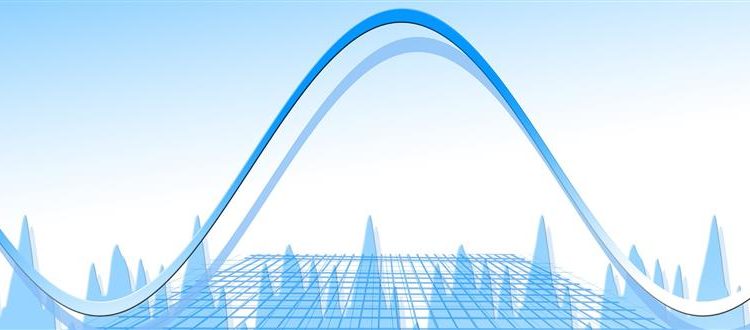
When scientists conduct experiments, they want to be able to gauge how results are predicted—their simple hypothesis. More specifically, they study conditions that will test what they call their “null hypothesis.” A researcher’s null hypothesis is a statement asserting that a variable has no effect. It is the opposite of the simple hypothesis and is what is tested.
What is a P-Value?
We test the null hypothesis because it can be proved false with a single exception. For example, if we see only black horses as we drive through the country, we might make a statement that all horses are black. That would be our null hypothesis. It would take only one horse of a different color to prove our null hypothesis false.
A “P”-value (P = “probability”), otherwise known as an indication of “significance,” is a means by which we show relevance to predicted outcomes. It is a calculated value based on study group size and data. Although most often used to gauge significance, some scientists suggest that P-value is not reliable.
Reproducibility Crisis
One of the main requirements of any study is that it be reproducible. Researchers must include a detailed methods section in their papers, report the number of subjects, and explain how their results were analyzed. These provide validity to the study. Unfortunately, according to Nature, we have a major “reproducibility crisis.” According to a Nature survey, more than 70 percent of respondents could not duplicate others’ experiments. Even more interesting, more than one-half of those surveyed could not duplicate their own results!
Sixty percent of respondents cited the pressure to publish and the lack of complete disclosure of results as the main contributors to the decrease in quality-control standards. Whatever the reasons, scientists are taking a hard look at the trends and discussing how to change them.
Statistical Significance
According to an article in April 2017, P-value is a measure of the strength of the evidence produced in a study. It is supposed to determine whether variables have an effect on an outcome. In most cases, the minimum P-value for statistical significance is set at 5.0 percent (i.e., P = .05). A value lower than this indicates that the results of the study might not be from chance. These are generalities; however, there are some misconceptions about what P-values actually represent.
According to another article, P < .05 does not necessarily mean that the findings are relevant. The outcome must have some kind of impact. In a clinical trial on a new drug, this might mean a change in patient treatment. It is also wrong to presume that a 5.0-percent P-value means that the results would occur only 5.0 percent of the time. In real cases, other variables might be introduced that cannot be observed. P-values are relevant for only the data or variable observed in that particular study.
Why set significance threshold at 5.0 percent? After all, the value doesn’t necessarily mean that something is true. It merely provides a level of probability for a true or false null hypothesis.
Issues with the Standard P-Value
In an effort to ensure a specific P-value, some researchers resort to “P-hacking.” In P-hacking, the researcher omits data or performs unnecessary statistical tests to “force” a specific P-value. Unfortunately, this practice of “cheating” is on the rise.
Why is this important? It is important because study results can have major consequences. For example, the results of a clinical trial on a new drug could be positive or negative. The null hypothesis would be a very important part of this type of study for obvious reasons. If the results are not accurate, many lives could be at stake.
If we are going to continue to use P-value as a measure of significance, some scientists believe that it is time to redefine significance. They believe that reducing the P-value threshold would resolve some of the issues with poor reporting and data manipulation.
Changing Thresholds
Those involved in research worry that the papers are filled with inaccuracies. To resolve these issues, a group headed by Dr. D. Benjamin (Center for Economic and Social Research and Department of Economics, USC, Los Angeles, CA) suggests that the P-value threshold change from 5.0 percent to one-half of 1.0 percent, or .005. This would be quite a change!
In their article to be published soon in Nature Human Behaviour, Dr. D. Benjamin et al. claim that a P-value threshold of 5.0 percent provides only weak evidence. They also suggest that a P-value between 5.0 and 0.5 percent be recognized as “suggestive,” not concrete evidence.
Pros and Cons
Those who support this change say that it would help reduce P-hacking and false-positive results. This would help resolve the ongoing problem of lack of reproducibility. In fact, many who do research studies in genetics have already adopted a similar idea with good results. Another positive outcome would be that the costs of having to follow up on false-positive results would decline. More results would be deemed as valid.
Other scientists are not as eager to agree. Some worry that having any type of absolute threshold creates a potential for error and data manipulation. Researchers working in the field of drug testing see this as driving up the cost of the clinical studies because larger sample sizes would be needed. Still, others are abandoning P-values altogether and using more sophisticated tools for statistical analysis, such as Bayesian tests.
You must always remember that your paper is only as good as your study protocols. Your results are only as good as how you conduct your study and analyses. Whether you continue to use P-values to determine significance, it is of utmost importance that your findings be true and that you do not manipulate your data for any reason.